
Understanding trade-offs in decision-making
Imagine you're a BI practitioner trying to understand how people decide which TV to buy. They'll probably consider the cost of the TV, various specific features like size or display resolution, and – in this interconnected day and age – they might also consider whether it has extra functionality to connect it to the internet, making it a 'smart TV'.
As a BI practitioner in this situation, you might be tasked with finding out how people make trade-offs when considering multiple desirable features – e.g., 'Do I want the TV with the highest screen resolution, or the one with better colour technology?' Or, you might wonder how much people would be willing to pay for a specific feature – e.g., 'Would I be willing to pay more for a smart TV with a cyber security label, compared to a cheaper, unlabelled, one?'
These kinds of trade-offs aren't confined to choosing TVs, or even to the domain of shopping. For example, when choosing a course of study, people might need to weigh up features like scholarship options, length of study, and starting pay. But the structure of the problem is the same in all cases: People are choosing among multiple options, by weighing the options' features against each other. And so, the research question is the same: How does feature X influence people's choices?
The advantages of DCEs
Last year, BETA added a new method to our repertoire, specifically for these kinds of question: Discrete Choice Experiments (DCEs). Used extensively in market research and economics, DCEs have a number of qualities which make them great for analysing and understanding the kind of complex choices people make when they buy a smart device or choose a course of study.
- In a classic randomised controlled trial (RCT), you are usually limited to testing a small number of variations on features in your interventions. Even a factorial design only allows a small number of features to be varied before the sample size becomes too large and analysis too complex. Using DCEs, you can test a higher number of features, with more variations (e.g. 1 to 4 star ratings), and still not have the analysis get out of hand.
- DCEs provide a direct measure of how people make trade-offs among features. The most common trade-off is 'willingness to pay', or 'how many dollars would people trade for feature X?' But you can also look at the influence of – for example – a cyber-security label compared to the influence of premium product features like higher resolution on a TV or colour screen on a smart watch.
- The choice participants face in a DCE is familiar and simple to understand as it mimics a typical 'online shopping scenario'. Although participants are usually not spending any actual money during a DCE, the choices made in this setting appear to have pretty good external validity.
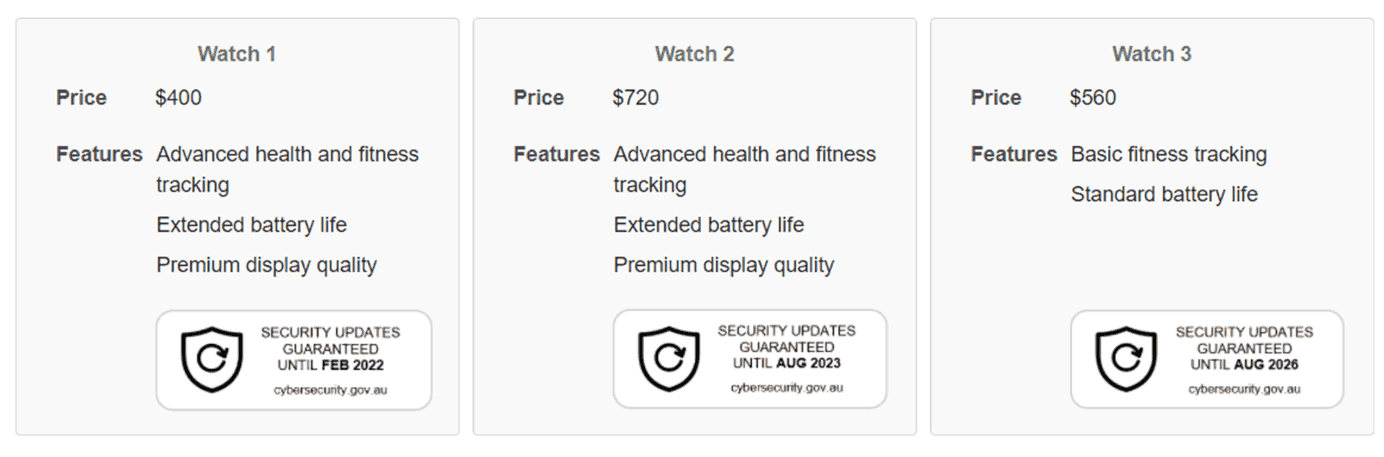
The disadvantages of DCEs
With all these advantages you might be wondering – what's the catch? We found there were three main disadvantages of using DCEs.
- The DCE technique assumes that researchers are able to select and capture in a simulation the features that matter to consumers in the real world. That is, if I ask you to pick between three washing machines with different costs, programs and energy efficiency, in a DCE I'd assume you were only taking those product features into account, not being influenced by something external. This might be a realistic assumption when it comes to washing machines, where I can be relatively sure of capturing the relevant features in my examples. But it's less realistic for choices that are driven relatively more by individual or personal factors, such as values, emotions, or appetite for risk.
- DCEs are fairly complex to set up and analyse. There are software options that can help but it's definitely a steep learning curve.
- Despite the name, a DCE is not actually an experiment, and you cannot draw causal inferences on the basis of the data it provides. Instead, what you get are associations between an option's features and participants' choices. For example, higher prices are associated with a lower likelihood that a device will be chosen. Stronger cyber security is associated with a higher likelihood. And so on.
Conclusion
Overall, we found that DCEs were a great technique for a couple of recent projects. Thanks to a DCE we were able to estimate people's willingness to pay for cyber security labels on smart devices, for example smart watches and smart TVs. In a separate project, the DCE helped us demonstrate that scholarships and ongoing employment could encourage high achievers to go into teaching. And next time we need to understand how people make complex decisions involving many trade-offs, we'll consider using DCEs again.