Transcript
Susan Calvert
Hello, welcome to BI Connect for 2024. Hi, I'm Susan Calvert, I'm the Managing Director of the Behavioural Economics team of the Australian Government, which you'll know as BETA.
We're delighted to be showcasing some really innovative and impressive behavioural insights projects today from right around Australia. Thank you so much for joining us. The 1200 of you that signed up is testament to the growing BI community in Australia.
To provide the Acknowledgement of Country and to open BI Connect, I welcome our Secretary, the Head of the Department of the Prime Minister and Cabinet, the fabulous Professor Glyn Davis.
Professor Glyn Davis AC
G’Day and let me acknowledge the traditional custodians of the many lands in which we meet today right across Australia. I'd also like to welcome and acknowledge some Aboriginal Torres Strait Islander people who are joining us for this conversation. We are privileged to be on your country, to benefit from the continuing culture and care you bring to the Australian community.
I'm delighted that PM&C is hosting BI Connect and I want to start with a shout out to BETA, the Behavioural Economics Team of the Australian Government, and to you all, the thousand or more participants who work to generate and apply evidence that's critical to good policy and effective programs. It's hard to think of many policy areas where a better understanding of a human behaviour is not a good thing. The ability to understand how people interact with policy and programs is important to ensure that we deliver sound advice to the government and that we best serve the Australian people.
Over many years, BETA and behavioural science teams across the APS have helped Australians navigate government processes, improve access to services, tackle workplace challenges, simplify financial decision making and support effective policy design. I'm really encouraged to see behavioural science units using rigorous research methods and randomised control trials to understand what works and what does not.
The BI Connect Conference also highlights the value of close working relationships between the Australian State and Territory governments, between academics from universities across the nation, from practitioners from industry, and from members of the public with an interest in behavioural science. This is a great opportunity to share new knowledge and research, to learn about emerging methodologies and to explore solutions in a cross-sector forum. So, the 2024 BI Connect Conference will showcase behavioural insights in three important areas.
Today's sessions commencing the conference, we'll look at innovative methodologies being used by BI practitioners to support the application of behavioural science in public policy.
As the field evolves, exposure to novel and emerging behavioural science methodology can spark creative initiatives. It can uncover new directions of thought. The next session focuses on financial well-being, a topic that is timely in the current economic climate as Australians experience cost of living pressures. And the final session turns to working at the margins and will include speakers who've worked with under-served and hard to reach population cohorts. From my time at the Paul Ramsey Foundation, I recognise how important it is to break the cycle of disadvantage and to acknowledge our moral responsibility toward others. The research discussed will showcase the efforts to engage our most vulnerable and disadvantaged citizens. And the session will remind us that inclusive, ethical research, empowering diverse communities and unlocking hidden perspectives are priorities always worth pursuing.
From the outset of the conference, I encourage you to take advantage of the experts who've gathered. We all know that great teaching comes from conversation, so let me encourage you to engage actively in the Q&A and to think about how the knowledge you gain from this discussion can be used to improve the lives of Australians.
So it's a great honour to open the Behavioural Insights Conference series, BI Connect 2024.
Susan Calvert
Thank you so much, Secretary. We're very lucky to have a professor as the head of Government. He's such a strong supporter of the value of applying a behavioural lens to inform public policy solutions. At BETA, our mission is to improve the lives of Australians by generating evidence from the behavioural sciences and finding solutions to complex policy problems, and we're always looking for innovative ways to achieve this and to share our learnings with our colleagues.
Over the last year, BETA has tested some new technologies to support our project work. We've trialled the use of large language models with the aim of speeding up our literature reviews and to be honest we've had mixed success with that. But we have had fantastic success using eye scanning technologies to track how customers view information, and we've used this as a basis for designing better energy bills.
We've also trained our data team in machine learning and have automated our integration of huge government data held assets. We for example, to study whether cybersecurity job ads are written in a way that discourages women from applying, we searched through millions of records. And more and more, we're using apps and decision support tools to improve access to services for Australians.
In today's BI Connect session, we'll learn about some truly innovative methodologies and behavioural science. Our presenters are from three organisations known for their leadership in innovative practices, and the format today is three short presentations followed by questions. And we also want to hear from you. So please put any comments, innovative ideas and questions you have down in the Q&A section, and we'll do our best to answer them as we go. To do that, you click on the Q&A button in the top right corner of your computer screen.
But it's great for us to be able to welcome the first presenter today, and that is the fabulous Doctor Bowen Fung from the Behavioural Insights Team. Bowen has completed a PhD in Cognitive Science at the University of Melbourne and he's worked as a postdoctoral researcher at the California Institute of Technology. He is now a senior research advisor at BIT and works on projects in a diverse set of fields. He's been involved in the development of a number of machine learning tools that are widely used across BIT, and I can't wait to hear his presentation today. He's talking about two novel methods using generative AI, which work to enhance existing behavioural science approaches. It's a very warm welcome to you, Bowen, and over to you.
Dr Bowen Fung
Thanks very much, Susan. So, I'm sure you're all aware at this point and feel maybe that AI hype has reached a bit of a, a fever pitch. But I'm here to try and ground the conversation a little bit and talk to you about two novel methods that we've been using at BIT that we find quite useful for behavioural science. They're just really piece meal technologies and methods that we've been using. But I really think they do showcase the creative ways in which AI stands to impact behavioural science. And so, at the very end of the conversation today, I will talk a little bit about deliberative democracies.
So just very quickly, I'm going to talk through these two methods, PersonifAI, which we use to generate personas, simulated eye tracking, which we use to essentially create predicted salience heat maps. And then again, as I mentioned, deliberative democracies.
So when we're talking about PersonifAI, we're really responding to a lot of range of clients and a range of different people that we work with increasingly want to use behaviourally informed solutions that are really tailored to the different members of the diverse communities that they serve. We've seen the kind of off the shelf solutions breakdown when we're trying to apply them across a population of different people. And so, what we really want to do here is look at different ways that we can kind of serve more diverse groups and make sure that the interventions that we use are bespoke.
The idea of segmenting the market and generating personas like this isn't really new at all. But relying on the data that clients often have about the communities, things like age, gender, household income, education levels, often make for personas that are kind of stereotypical or they might end up being quite irrelevant to the behaviours that we're actually interested in. So, while there are established approaches to gathering more meaningful data to kind of generate personas that we can use to try and characterise different communities, they can be time and resource intensive. So, what we've developed here is essentially a method to try and shortcut this and give us some informed insights around the different personas that might be making up different communities.
So when we talk about PersonifAI, we're actually just talking about a custom GPT LM. So, what we've done is we've designed this very carefully to try and generate evidence-based personas centred around a specific target behaviour. What this gives us is personas that are differentiated by their values, motivations, biases, barriers, and enablers. And because they're specific to a target behaviour, what we can do is we can use these personas to kind of generate really bespoke solutions or even universal solutions that work across all of them. What we've done is just to ensure that PersonifAI works properly is that we make sure that it draws from the scientific literature about behavioural science, behavioural economics, psychological motivation, and different cognitive biases.
We also draw from insights from marketing and communication studies. This is especially the case if we're kind of asking about different messaging strategies that we might use for these personas. We draw from UX and service design principles, and that might be especially the case if we're thinking about interventions. And then it'll also draw from empirical data about the target behaviour that we might specify.
So how does this work in practice? Just as an example, here, we've got a scenario where we're trying to work with audiologists to figure out whether a new diagnostic tool would help them refer specific patients for cochlear implant evaluation. Audiologists are often busy, just like many kinds of medical health professionals, and this is the kind of key barrier to adopting any kind of new processes. And so, what we want to do in this scenario is we want to try and generate a behavioural persona for all of these different audiologists and clinicians around the target behaviour of adopting this tool for clinical practice.
So all this really takes is to provide PersonifAI prompts like the one on the right here that we've essentially created this new assessment tool. I'm really just specifying that the target behaviour that we're interested in is getting audiologists to use this tool in their clinical practice. What we then ask is whether some, if I can simply generate personas.
There's a lot of information on this page, so I'm not going to go into detail here, but this is the kind of example that we expect to see generated by PersonifAI. It will kind of default to around four personas, which is a kind of good amount if we're just trying to essentially characterise the main components of a different community. And what we've got here as the first persona is the cautious validator. So, this persona is kind of reflecting the idea that this is a clinician that really values evidence-based practice, but it's really cautious about applying new tools and making sure that they're robust before anything else happens. You can also see that it breaks down the motivations of this persona, the barriers, the enablers, and the cognitive bias he's inherent with this one. And there's a range of the other three personas kind of alongside that as well, which you can read through if you have time there. What we can do then with these different personas is we can have a look at them, we can validate them, we can use these for just kind of initial insights. I find this very, very useful when exploring different or kind of new domains that I'm not used to. It's a very, very quick way to kind of get a good understanding of the landscape. But we can then also go in and try and use these personas to develop some bespoke solutions.
So one opportunity here is to try and develop a universal intervention which can take into account all of the different characteristics within those personas. So in this example here, what PersonifAI has come up with is an interactive online, an online continuing professional development activity to promote knowledge and the use of this tool, it will tell you all of the rationale behind why each of the kind of persona types would want to be involved in such an event. And then it will even tell you kind of different communication strategies that will work for each persona.
We can also do other things with PersonifAI. We can ask it to generate surveys with kind of associated scoring systems that might be helpful to kind of figure out which personas people belong to. And we can also kind of play around with these persona groups and ask it to reconsider or redivide them based on other empirical data that we might be able to feed it. So PersonifAI is a really good way of kind of shortcutting some of the kind of more arduous qualitative testing. Again, it's not a replacement. If we want to do this kind of outreach, we absolutely should be doing qualitative participatory methods to kind of engage with communities and understand them properly and to validate that the personas that PersonifAI is generating actually work.
Next, I'm going to talk about simulated eye tracking. So we heard from Susan that BETA has been kind of working a little bit with eye scanning, using kind of web tools to understand exactly how people are paying attention to different visual resources. What simulated eye tracking does is to kind of shortcut this and provide a prediction of what we're going to see.
This is really important because understanding the salience of objects, basically how much they attract attention or the salience of information is really important to ensure that we can communicate effectively to people as well as to help people make decisions that are good for them. This is reflected in the way that a lot of strategic redesigns of visual communications often make information just simply more attractive. But the question here is like, how do we know what's actually attractive? People have good kind of common sense design intuitions for this kind of thing, but sometimes this is quite subjective or sometimes when we're kind of designing information, we kind of rush it out and we don't think about it too much. So the question here is, can we generate some objective evidence that something, information, communications, visual material will actually attract attention?
Eye tracking, as we know, is really critical for this. It's involved in memory, forecasting, decision making. But the key thing here is we want to be able to provide some objective evidence that something is attractive, that something will engage an audience. The issue here is that traditional eye tracking is quite resource intensive. So I don't know if we've got any kind of psychologist in the audience who worked with traditional eye tracking before, but it's quite fiddly. It requires a lot of expensive equipment, intensive data collection with participants and there's kind of complex analysis processes as well that you have to use with this and sounds like it might have had some experience with this recently. So in some cases when you kind of have a, you know, low priority piece of visual information that you want to push out, it's simply not worth the effort in kind of rolling out a whole eye tracking study. This is just an example here that I pulled from the Internet of kind of what eye tracking will look like for any of you who aren't familiar with it.
So what we can do instead is that we can apply AI model to basically generate the predicted heat maps of where we think that human attention will fall on visual materials. So you can see this on the example on the right where we pulled some visual materials from the U-Save campaign that the Ministry of Finance from the Singaporean Government have been using. We can essentially run this through the algorithm and it will display this salience heat map and tell you where critical information should be placed, what's currently in the visual information that's drawing people's attention.
And from this we can identify things like where they might be kind of distracting elements or where design can be kind of reshaped to better inform those communications. This works on pretty much any visual material, any static visual material. So we can use this for websites, we can use it for screenshots, or we can even use it for photographs that we take in the field. And what the machine learning algorithm does kind of at its base level as it analyses the low level visual features. So things like contrast, colour and shape. And it also looks at the high level visual features. So in the example on the right, you're seeing that this cartoon face is actually attracting a lot of attention. And we see this a lot in the attention literature is that human faces often attract quite a bit of attention.
We can also use this for kind of slightly more run-of-the-mill communications like bills and letters. This doesn't contain heaps of visual information, but it still works quite well and you can see here how attention is being paid to the kind of final dollar amount as well as some of the key elements here. Following that kind of F-shaped design principle where people's reading direction informs this.
We can use simulated eye tracking for a number of different things in the kind of behavioural science toolbox. We can use it to help inform the iterative design of visual materials where we're testing these things. We can make sure that we can improve accessibility in online environments. We can make sure that critical information is prioritised. Like I mentioned before, we can make sure that distracting content is minimised. We can compare different interventions. If we've got candidates visual interventions, we can compare them to see how much each one is engaging. And we can also just sense check things that we want to sense check before we kind of push them out into the real world.
I wanted to mention that while simulated eye tracking, like PersonifAI, can provide really kind of valuable insights as a bit of a shortcut to try and look at paternts of visual attention, there are some real limitations to this. So for start, it can't capture dynamic aspects of eye movement like kind of real eye tracking can, and it only really provides a prediction of how a person might be looking at a document.
So while the algorithm is really well validated, it only presents an average of how people will be viewing information. And there might be differences in eye movements, visual impairments, external factors like the lighting that people are looking at visual materials in. That means that the predictions aren't accurate. And these are the kinds of types of issues that I think that a lot of policy makers might not be aware of because they capture a little bit of nuance. And even though these innovations that I've just talked about can help in theory, make behavioural science more effective, what we want to do is we want to make sure that policy makers that are using these tools and the public that are having these kind of tools used in the background understand and accept them. So I guess the hype that AI has created has created a lot of gaps in understanding. So these are gaps in ethics, gaps in between the goals of executives and what the public wants. And so to really ensure that that these types of generative AI tools and other AI tools have positive impacts on our lives, the public should really have a say in how it's created. Otherwise it risks causing harm when it really should be improving outcomes for them.
So in this very, very final part of the presentation, I'm just going to touch on this by showing how deliberative democracies can bridge some of these gaps between policy makers in the public and ensure that there's some agreement on how technology should be used. So the example I'm going to point towards here is an instance of deliberative polling. So this is with the company Meta, we ran what they call a community forum with about 1500 participants across the US, Germany, Spain and Brazil. So a real global effort.
And what we did here is we had participants basically deliberate and discuss with each other within their own countries some of the topics around the principles that should guide how AI chatbots offer advice and guidance and how they should be developed responsibly. What happens in these cases is that we will define themes, we'll develop a lot of resources that are really clear to make sure that there is a baseline level of understanding in all of the participants. And then we'll allow the participants to kind of discuss together with themselves and also with experts to kind of understand and then eventually kind of vote or poll on a series of different proposals.
What we then do is we take the samples of these discussions and we explore participants’ mental models of these. We figure out their priorities, their perceptions of the process itself. And we can translate these into actionable recommendations for, in this case, tech companies so that they can incorporate these informed public opinions into their governance structures.
So the kinds of things that came out of this particular instance of a deliberative forum were that human rights should be the baseline of acceptability for chatbot perspectives. This might be kind of a bit of an obvious thing that there are certain things that participants want to prioritise like global credible sources. So things like peer reviewed academic articles, other things that they'd like chatbots to pull from that the public really want to see experiences that actually enhanced by personalisation. So this is something that again wouldn't necessarily be intuitive, but there was some conditional support for tech companies basically being able to personalise chat bot experiences based on users past online activity. There are a couple of other recommendations that came out of this and we're looking at the moment to see whether Meta is going to take us on in their development. But it's an informed way to bridge that gap between policy makers and people who are developing these tools and the communities that are actually going to be interact with them.
Overall, this is the third, I think, deliberative process that we've run with Meta so far, but we've also run a number of similar deliberative processes around the world with the governments and other organisations and a range of topics so on things like misinformation, obesity and governance. But I think AI is one area that can be very useful at the moment since there is really such, such a huge surge in content around AI as huge surge in new tools and innovative methodology.
That's it for me. I really appreciate your time here. And if you want to get in touch, please contact me at Bowen Fung at BI Team. Thank you, Susan.
Susan Calvert
Thank you so much, Bowen. That was absolutely fascinating. I just want you to know that I've bought my evidence based persona to the conference today. So I'm keen to see the other sides of myself as well. There's some excellent questions coming through in the Q&A, so we'll get to those after other presenters. And Bowen, you might try and answer some of those as we go.
So next up, we have Behaviour Works Australia and our presenter Jennifer Macklin leads circular economy behaviour change research at BehaviourWorks Australia. And that's all within the Monash Sustainable Development Institute. Jennifer has recently been delivering research collaborations with government into the circular economy to develop effective policies and programs for households, organisations and system-wide futures. And so welcome to Jennifer. We're really looking forward to your presentation.
Jennifer Macklin
Thanks Susan. As Susan said, it’s really great to be here to present some of the work from Behaviour Works. For those of you who don't know us, we are a research centre at Monash University and we have ongoing research partnerships with a number of public, government partners, businesses, civil organisations. We also do individual behavioural science projects and a large range of educational training. We work across a whole range of areas and recently we've been exploring actually bridging behavioural science and system thinking.
And we've been drawing on inspiration from Joanna Hale and colleagues coming out of the University College London Centre for Behaviour Change, who've actually pioneered an approach that we find really interesting. And this is our kind of adaptation and innovation of that technique that they've developed. And the reason that we came to this is because often in behavioural science, what we're trying to do is to take a big problem and to kind of narrow it down into something really concrete and tangible that we can actually make a difference about. So we might look at a problem and all of the behaviour that are contributing to that problem, and then pick one particular behaviour and then for that behaviour, understand all of the barriers and then pick a few barriers that we're going to target through intervention. And then again, with all of those ideas that we have, the interventions, pick something that we can actually take to trial to develop effectiveness. And this is really good at producing something that has the greatest chance of being effective for that particular part of the problem, but it is only tackling one very narrow part, often of a very big problem. And so behavioural science has in, in some regards started to, to understand this difficulty in trying to use our tools and techniques to, to really tackle those big complex challenges. And so we're quite interested in the idea of system thinking, soft systems approaches, particularly system mapping, as a way that can actually integrate with behavioural science. So actually trying to capture all of the elements of the system and keep all of that complexity in the work that we're doing.
And in the past, we've seen and used a number of different types of system mapping approaches. We've used actor mapping, which lays out all the different stakeholders related to a problem. We've done causal influence mapping, causal loop diagramming, where we actually say what are all the different things that are contributing to a problem. But we've struggled a little bit with integrating these processes into a typical behaviour science process. For actor mapping, it's difficult to kind of represent in an active map all of the different ways that these stakeholders interact and the different way they influence each other. And it's not always clear exactly what we might want a target audience to do differently from the map. With causal mapping, it's difficult to understand how we actually change a particular part of this. How do we intervene, how do we actually marry up our behavioural science process to a particular part of a causal map of a problem? And so behavioural system mapping is kind of a conglomeration of these two. It's actually looking at mapping the behaviours of actors in a system and understanding their influences.
And so in one sense it's really literally having a behaviour and then another behaviour and understanding the relationship between those two behaviours. And then you can go another level to also add influences on behaviours into your system map. But at a simple level, what you end up is with a map of a number of different behaviours by different actors or audiences and how they influence each other to either contribute and create a particular problem or perhaps to actually implement a solution. And what's really great about this is that when we do this behavioural system mapping, when we pick a particular part of the system to intervene, it's really clear for us to see who and which behaviours need to change. And then be able to actually apply our behaviour science process to having identified a behaviour. And then to work out, you know, what's going on to diagnose, understand that behaviour and then develop interventions.
And so at a high level, behavioural system mapping is really just identifying the behaviours that are, you know, causing a problem or might contribute to a solution, and then mapping the connection between those behaviours. And that's really it, you know, sort of it's as simple and as uncomplicated as that.
And as an example of how this works in practice, one of the pieces of work that BehaviourWorks has recently done was looking at circular consumption. So how can we actually reduce the material footprint of the use of goods in Australia's economy?
And we had identified a number of behaviours and we really wanted to understand how these behaviours actually related to each other. And so we were looking for the connections between these behaviours. And we know that two of these behaviours directly reduce material footprint. If people make do without a new item, or if people buy an item second hand instead of new, we're not bringing new products into the economy, so we're reducing the material footprint. But it's not quite that simple because if we want people to make do without new products, that means that they actually need to keep using items that they already have. And in order to keep using items, they actually need to have bought items that are going to last a long time, that they can keep using them. They're probably also going to need to get those items repaired at some point, as, you know, different types of functions, you know, start to break down or as technology advances, they might need to upgrade in order to do that again, they need to have bought items that actually have that functionality built into them that are repairable or upgradeable.
If we're looking at this behaviour of like, you know, getting people to make do, that purchasing new, what we actually realise is what's dependent on a number of other behaviours that that person needs to have already done and been able to do. There's something like sourcing items second hand. When we look at it, what we realise is that for someone to be able to get something secondhand, it actually depends on someone else actually making an item available that they no longer want anymore. But at the same time, for someone to be able to pass on an item, to sell it or to donate it, there needs to be an item that's going to maintain its value. So it needs to have been built to last so that it's going to keep its value and be worthwhile for the next user. But also we have a bit of a dependency here. So not only does someone who wants to get an item second hand need someone to be passing on an item, for someone to successfully sell or donate an item, they need other people to want that item.
And so we can start to see some of the dependencies here. If we're trying to work out where we want to intervene in this problem, which behaviour we want to target to try and change, we can start to see the complexity and the dependencies between these behaviours. So how does behaviour system mapping work with a typical behaviour science process?
At BehaviourWorks, we have quite a detailed method, but we've identified that our method and similar methods from other behaviour science organisations typically kind of have, you know, five key stages. The first one is to kind of really understand the problem or define the goal, then to identify and prioritise particular behaviours that we want to actually change, to explore the drivers and barriers to those behaviours, select and design relevant interventions that will target some of those behaviours and then test and evaluate. And you can actually use behavioural system mapping right at the start for defining the problem or the goal and then use it to identify behaviours. As I said, you know, we saw that to pick which behaviours you might want to actually target to understand those system barriers. So not just what's internal to a particular person, but how's their behaviour dependent on past behaviours or behaviours of other people dependent on other actors in the system or other system constraints. You can then use it to actually test an intervention by saying if we intervene in this part of the system, how does that impact the rest of the problem or the rest of the solution and to evaluate how effective an intervention has been.
You can also pick it up at any particular point in a behavioural science process. So if you've already actually identified a range of behaviours, you can use behavioural system mapping to actually identify the connections and then to consider system influence as one of the priorities when choosing a particular behaviour to target. If you have a target behaviour, or perhaps you've been given a target behaviour for those who work in sort of program areas of government and large organisations, you might be familiar with the experience of being told to develop a program or to fix a particular problem. You can actually use system mapping to confirm if that is the correct place to intervene and use that to refine or perhaps advocate back up that there might be a different way of approaching a particular problem. If you are looking at identifying barriers, you can make sure that you've actually identified all of the system barriers, the broader sort of more intangible things that might be impacting on whether or not people are performing particular behaviours. If you've got potential interventions and you're trying to choose between them, you can actually start to see how many of these actually more transformational interventions, how much of these, how much will each of your interventions actually flow through and transform a broader system as opposed to just the particular behaviour that you're looking at. You can also make sure that it won't have any kind of side effects when you're looking at your system. And finally, once you've done a behavioural science intervention and you've done an evaluation, you can also actually use it to clarify what you achieved versus what you should expect to have achieved given the system that your intervention is operating in, which can be really valuable for actually more accurately understanding the impact that you've had and articulating and explaining if you've got an intervention that doesn't appear quite as successful, you'll be able to articulate if that's actually something to do with the intervention design or if that's something to do with the broader system constraints that your intervention is interacting with. So really, really valuable throughout the whole behavioural science process.
When you're doing behavioural system mapping, there's a few decisions that you need. The first one is how you're going to frame your map and you can take either kind of a problem or a solution focus. So if you're doing, if you want to focus really on a problem, you can do a current or a problem state map. So you're mapping out what is going on now, who is currently doing what, and how is that influencing the behaviours of other people in the system. And that can be helpful when you've kind of got a big problem and you are looking for one part of the problem that you might be able to do something about with the particular resources that you have available. The other approach is to take a kind of a solutions perspective and produce a future or an ideal state map, which is actually mapping out in an ideal state in the future, what would everybody be doing if the problem had been solved, if a particular goal had been achieved. And this can be really great for developing larger programs, longer term programs of work, or when you're actually trying to align the efforts of a whole number of stakeholders because you can put together sort of a, a broader road map of how to achieve, you know, a particular system transformation or, or future state.
And with tools similar to most types of system mapping, there's a range of tools that you can use. You can do their physical tools like post it notes. You can put it into drawing programs like PowerPoint. You can use Miro, you know, or, or other sort of unlimited boards, you know, to really move things about. Or you can use dedicated mapping platforms like Kumu, which is one that we adopted. The final kind of key decision when you're thinking about integrating behaviour systems mapping is the degree of participatory development of your map. To what extent are you going to engage other stakeholders, including people experiencing the problem, people who might be affected by the solution, people who might be able to help develop and implement interventions, people who have, you know, done previous research. So what experience are you going to bring these people together in doing the map. And BehaviourWorks has actually had experience in different levels of participation. So we've done three recent detailed behavioural system maps, one around circuit consumption, which is kind of that example I touched on before, one around home energy efficiency and one around cybersecurity in a particular industry. And we have actually used different levels of participatory development in each of these depending on the resources that are available to the program, our access to the stakeholders and what existing insights we already get given from stakeholders. But you can have stakeholders help in identifying the behaviours that are going into your map. You can have them help articulate the connections between those behaviours and kind of develop sort of like partial maps, you know, for particular areas that they're familiar with. You can actually have them help connect into the overall map, although that synthesis is particularly challenging. So similar to UCL behaviour, exercising to do the connection part ourselves and obviously presenting the map back and validating and refining that map with participation with participants.
So just a couple of quick examples to get a sense of what behavioural system maps can look like. One of the maps, as I said, mapping exercises that were actually part way through at the moment is around home energy efficiency. And what we're doing at the moment is kind of building partial maps. So we're looking at individual kind of target behaviours and we're actually mapping out all of the behaviours that are actually implicated by a particular behaviour. So target behaviour of having household install solar PV, what are all the behaviours that need to happen across all of the different actors in order for this, you know, ultimate behaviour to be reached. And so we're, we're mapping that out across multiple behaviours and that actually allows us to see which of these behaviours are more or less dependent on other actors, which behaviours actually require more or less pre steps by our target audience, the home owner. And eventually we'll also be able to join these together to actually see other connections between these different behaviours. Like does install installing solar PV systems make you more or less likely to install non solar renewable systems?
Does doing one actually make it easier to do the other? Are there crossovers where some actors are involved in multiple behaviours?
We've also done a really technical behavioural system map in the area of cybersecurity where we use some of the more technical approaches to mapping. We actually mapped both the behaviours and the influences. We identified both positive relationships and where things actually improve a particular situation, but also negative relationships. And we actually looked for kind of loops within the system where we actually see what we call a balancing loop, which is where something positive is happening, happening, but it's being mitigated by something negative.
And so it's kind of a little bit locked in stasis. Or where we have amplifying loops, which can actually amplify problems. So things that can continually contribute to each other, kind of negative feedback loops or amplify positive aspects, what we might call like virtuous cycles, where if you could get one part of the cycle to happen, it actually improves the whole range of things sort of dynamically ongoing.
And our final map was around safer consumption. So I showed you just a couple of the behaviours before as an example, but we mapped together 130 behaviours across a range of different stakeholders across the economy to understand how we could reduce material footprint for consumption. And this was really interesting because our original task was to try and understand how to get consumers to more responsibly purchase and use materials in order to reduce material consumption. But what we found in the early stages were the system constraints were so great that it didn't make sense to necessarily target consumers as our audience that we actually potentially needed to look at other stakeholders, other audiences in the system first to actually enable consumers to eventually be able to adopt the ultimate behaviours.
And we used Kumu to develop the map. It's an interactive online platform and it actually has some mapping metrics built into it. And we were able to use some of those metrics to actually understand which behaviours in this system actually have this greatest system reach. So if we could get a particular behaviour to happen, how much of the system could it influence? And we actually identified that there were kind of like four key areas of change within the system that could really actually have a major transformation impact across the system.
And you know, the one that came out, you know, most highly government's mandate, minimum design or import standards for products actually affected the consumer's ability to do all of the behaviours that we wanted them to do so it's this really foundational behaviour. But we could also identify these behaviours across a range of different stakeholders. And for some reason you can't see the stakeholders on this slide.
But we identified kind of priority behaviours for consumers, for designers, for producers, for retailers, for third party service providers, for the community sector, for NGOs and advocates and for government. And so this really enables that kind of building a coalition to be able to say if we want a circular economy in Australia, what are all the things that we need to change and where can different stakeholders in the system actually take action to have that greatest transformational effect. So just a few examples of the ways that we've applied behavioural system mapping and a few considerations if you're interested in the technique for the problems or challenges that you're looking at.
Susan Calvert
Thank you so much Jennifer, that was fantastic. I also wanted to introduce your colleague Melissa Hattie. And so she's also from BehaviourWorks and has been involved in this work and she'll be here to help answer questions at the end. Melissa's a practising health psychologist and works across environment, health and social and educational portfolios at Behaviour Works. Her research strengths extend across qualitative and quantitative approaches and she's previously worked in government, academia and private industry. So thank you so much Melissa for joining us.
Melissa Hatty
Thanks Susan.
Susan Calvert
Keep coming with the excellent questions online everyone. We're really appreciating that and doing our best to get back to them as quickly as possible.
But our final speaker today is Dr Jason Collins and he's from the University of Technology Sydney, where he currently teaches a behavioural economics course. Previously, Jason has co-founded and led PwC Australia's behavioural economic practice and he's built and led data science and consumer insight teams at the Australian Securities and Investment Commission that we know is ASIC. And more recently his work has focused on how behavioural economics could be better and on how financial services firms can improve consumer financial well-being.
So welcome Jason. We're really looking forward to your presentation. Over to you.
Dr Jason Collins
Thank you, Susan. So I'm going to open with a quick story about a competition held by Netflix and they offered 1,000,000 to the team that could develop an algorithm that [indiscernible] film ratings with 10% better accuracy than Netflix's own model. And this competition began back in October 2006. And by June 2007, 20,000 teams have registered for this competition, 2000 of those submitted predictions. Now, it took a while for a team to claim the prize. So ultimately in 2009, it was won. Unfortunately, the prize that algorithm was never implemented, but still massive participation in that.
We're now seeing competitions of this nature underpinning a lot of the progress in artificial intelligence. So many people date the genesis of the current AI boom to the success of a deep convolutional neural network called AlexNet back in 2012 in that year's edition of the ImageNet Large Scale Visual Recognition Challenge. And the question basically those algorithms are answering is can I correctly classify this image? Now Kaggle has industrialised the running of these competitions. Kaggle if you know was actually founded by a former Australian public servant. And in these competitions private and government entities submit a problem and data and competitors they compete to develop the best algorithm.
The math Olympiad competition that's on screen I took that screenshot a few days ago is running now and already 1754 submissions made by participants in this competition and we're also seeing informal competitions of this nature emerge such as the measuring of generative AI against standardised benchmarks.
So whenever we see a new version of Claude and that's what this slide here is, Claude 3.5 initial release, new version of Claude, ChatGPT, Gemini Llama, typically they'll release performance or measures of their performance against these benchmarks. And the approach underpins all these competitions, this benchmarking, it's known in the world, I guess in the world of sharing artificial intelligence is the common task framework. So it's researchers compete to solve a problem using the same data set, each measured against the same scale. And there are many benefits to this common task framework. Most basically there's a common objective measure of performance. We can see what is state-of-the-art and we can compare apples with apples.
So we're actually, I want to sort of look at two different, say, generative AI offerings and had at least one measure that we know is directly comparable. There's also some, some downsides to the common task framework that I'll, I'll come to later. But the question I'm going to ask is, is there a behavioural science version of the common task framework? And according to some behavioural scientists, the answer to that is yes, the mega study.
So it was first labelled a mega study in 2021. But the idea behind the mega study is to test many interventions in a single massive experiment. Don't test one intervention you get to control test 50. Observe the competition between those 50 different interventions to see which works best. Now, the idea of testing many interventions in this way has been around since before behavioural scientists, you know, put on their marketing hat and decided to call them mega studies. But the mega study has certainly increased in frequency over the last couple of years. There's five little shots that I've put on this slide. Now there's a fairly simple case for the mega study on why we should be thinking about that, this approach.
We've got a lot of studies out there, you know, some ways, a huge behavioural literature, masses of heuristics and biases that have been accumulated, all showing the effects of them on, you know, on human behaviour. You know, we have social norms, we've got framing, we have scarcity incentives and so on. And so if you're looking to change behaviour, which of those is going to be most effective? Right now we kind of use a bit of expert judgement because we can't look to the experimental literature to actually get a direct comparison. The typical academic paper will have just one or a couple of interventions tested against control and that's what gets published. We don't have this broader cross comparison happening and a mega study actually enables us to do that.
To illustrate, let me walk through the highest profile mega study, this was published in Nature. And in this mega study, Katherine Milkman, the lead author and friends, they tested 54 interventions to increase the gym visits involving 61,000 experimental participants. And in this experiment, members of a national gym chain, they were asked if they wanted to enrol in a habit building science based workout program. So those who signed up, they formed the subject pool and they were randomly assigned across these experimental conditions, including a control group and that control group basically received no further contact. So over the following 28 days, participants were subject to a bunch of interventions basically that involved varying mixes of messages and incentives.
For example, those in the social norm, high and increasing treatment group, they receive 6 text message reminders with content such as this: “trivia time, what percent of Americans exercised at least three times per week in 2016? Reply one for 61%, two for 64%, three for 70% or four for 73%.” And if they respond 1, 2 or 3, they receive a message back stating it's actually 73%. And this is up from 71% in 2015. They also received emails with similar facts, whereas those in the social norm low group, they received a message with a less rosy situation: “trivia time, what percentage of Americans exercise at least three times per week in 2016?” Numbers between 35 and 44%. And the response after they make their guess? It's actually 44%. As an aside, there doesn't seem to be any qualms about using deception here. Some of the interventions actually involved incentives. For example, there was an intervention called rigidity rewarded, where basically sticking to plans was rewarded. That intervention paid 500 Amazon points. That's worth about $1.79 every time they attended a planned gym visit and an extra 250 Amazon points worth about $0.90 if they attended the gym at another time.
So the headline results of all the interventions that are in this figure with the effect sizes and the 95% confidence intervals represented by the blue lines. So the blue lines sort of sit on the left side of that chart. Now, 24 of the 53 interventions were found to have a statistically significant effect over the control of no messages, increasing visits by between 9 and 27 percent. That equates to roughly, you know, .14 to .4 weekly extra visits over the control average of one and a half visits a week. Now that figure also contains some predictions made by behavioural practitioners, health experts and lay people as to which interventions would be most effective. And their predictions represented by the orange bars which sit further to the right of that diagram. They're sitting further to right indicating that those people grossly overestimated how powerful the intervention would be. And you kind of see that there isn't any relationship between their predictions and the actual ordering of those interventions and for the ordering the effect sizes for those interventions. And I'll come back to those predictions in a bit.
There was another mega study by the same authors released that same year, looked at messages to encourage vaccination. Here's one example message: “John, this is a reminder that a flu vaccine has been reserved for your appointment with Doctor Smith. Please ask your doctor for the shot to make sure you receive it.” Again, a little bit deceptive in that there's no reserved vaccine. But as you can see in this chart, that particular message of a highlighted flu dose was the most effective.
So you see that these medicines are giving us direct comparability across a vast landscape of potential interventions. But beyond giving the direct comparability, there's also some other nice features. So there's an economy of scale that comes with it. So even though an individual mega study, it's a pretty large exercise, could be quite costly on a per intervention basis, it'd be quite cost effective. Another thing that I really like about mega studies is that there's actually built in publication of null findings. So we get to see both the success, the successful interventions, and also the duds.
But what can you as a practitioner or a behavioural scientist do with the output of a mega study? Now, if you're that particular gym chain, that particular vaccination provider for which the mega study was conducted, you might scale the most successful messaging, the most successful interventions. But what if you're operating in a different context? So what? Imagine you're a gym chain with different customer demographics or a yoga studio. Imagine you're a university encouraging student attendance, a preventative health provider. So as we want to think about this, like what we could do in that different context, when you actually think about the fundamental problem that the mega study is designed to address, and that's the lack of comparability of interventions tested in different contexts. So mega studies are there because the context of two different experiments may be sufficiently different. That's not reasonable to ask which intervention is more effective. But if we can't easily compare across experiments in different contexts, what confidence can we have that moving the results of a mega study from one context to another, we'll see the same ordering of effect sizes in that different context. We're actually in a little bit of a catch 22 situation where the bigger the comparability problem that the mega study is seeking to solve, maybe the mega study results themselves may be actually less useful for application in other contexts. And ultimately this, this is why, you know, good policy, business advice is nearly always run your own experiment.
There's also a question about translating particular interventions into new contexts. Now, for those of you who have developed behavioural interventions before. messaging like you know, there's so many degrees of freedom that you grapple with visual design, precise wording, choice of medium, the timing. And the result is that it's typically not hard to come up with reasons why or why not an intervention will be effective for the reasons that don't actually relate to the particular phenomena or idea that's being tested. So just because a copy may not convey the concept, the wording could be confusing, You may not have written it very well. And this, this message on the screen here, this is an example from the first mega study on vaccinations. And this was the worst performing message of all, all of them and basically said: “it's flu season and getting a flu shot at your appointment is an easy thing you can do to be healthy”. So like when I first read this, I sort of like who are their copywriters? Because it's quite arguable that the poor, poor performance of this message gives you actually little information about the effectiveness of health messaging. If you did 40 different health messages, would you see different results? And this then comes down to this point, when you're trying to implement and interpret a mega study from one environment into your own environment, you've actually got those same implementation problems - you're changing wording, you're changing things and, and as and you're not quite sure sort of, you know, how much the implementation exercise is actually going to change the result. So again, points to, I guess the usual advice is to test in your own domain. A mega study, just like other experiments, isn't going to save you from doing your own testing.
And this brings me to possibly the biggest challenge with mega studies. So on the face of it, that mega study on gym attendances, it's a pretty big sample, 61,293 participants sounds pretty solid. But when you think that about it and go hang on, that's, that's sixty odd thousand people, they're divided across 54 interventions when you include the control. So that's not more than, many more than a thousand participants per intervention on average. And that relatively small number of participants per intervention means that we have low power. And for those of you who I guess I haven't done much statistics, power relates to the low ability, the ability, to detect effects that exist and to differentiate between interventions. If we have low power, even if an effect is there, we may not be able to see it. And the power problem you can see in the gym mega study where the largest effect size from that bonus for returning a missed workout, the effect size there was actually indistinguishable from around half the other interventions. So you could actually statistically say that it was better than the top half. And the mega study on vaccinations has the same problem. So though 19 interventions across 47,000 participants average boosted 21.1 percentage points, but the authors couldn't actually reject the null hypothesis that all 19 messages had the same effect size. So we had a mega study which effectively couldn't tell us what works. So not all mega studies have this problem, but they actually highlight the core issue we always grapple with as experimentalists. And that's as you increase the number of interventions, you reduce the power of your experiment to find an effect. So you basically, if you're going to add more and want to maintain the same power, you need to increase your sample size. So it's a trade off and sometimes you're simply better off with fewer interventions.
Now beyond the applied nature of mega studies, the question I always ask, and this is probably just getting on my little high horse here, is what mega studies offer to science. So this this flagship mega study paper was published in Nature after all. And to answer this question, let me describe a little experiment by Google. It got quite a bit of coverage at the time it became public. So when you visit Google online, they want you to click on advertising links. So what colour link is most likely to induce a click? And Google doesn't mess around when they deal with experiments such as this. So they tested 41 shades of blue in one experiment. And so outsiders, they ridiculed this experiment, they called it the 50 Shades of blue experiment. But that experiment yielded $200 million a year in additional revenue for Google. So the question in my mind is where do mega studies sit between this Google experiment, which is like a very highly valuable optimisation exercise but of limited scientific value? And a study designed to teach us something about how humans behave. And today the mega studies of like in my mind are sitting a little bit close to the, to the Google end of the spectrum. They're valuable for the task being optimised. So in terms of boosting gym attendance and the health effects in terms of boosting vaccinations, highly valuable, but they're not providing a lot of feedback yet into understanding human behaviour. So each interventions like within the experiment is backed by science. They're using empirical regularities found by past experiments, but we're not seeing mega studies to try and tie them together to give them backbone. Instead we're seeing domain specific horse races. So great for policy makers, business owners, perhaps less benefit for science to date. I'm actually asking a lot here. I'm probably being a little bit overly critical in asking for everything, but I do want to see behavioural science building understanding of what's going on. You know, I suppose we can't always have everything.
And I should say like this, like a theory isn't without costs for the behavioural practitioners. So I've already noted back to our gym effect size study that the predictions for were not very accurate. That's the orange bars on the right. The practitioners basically had no idea which would be better. And what that means is when behavioural practitioners are trying to advise you as someone who is going to go run a study, well, which are most prospective interventions to run? They’re actually not able to give you great advice. You're stuck throwing as many interventions against the wall as you can manage given your sample size. And if we had better theory, maybe we could winnow down options and have a higher powered study. So mega studies are in part a symptom of, of this failure.
So the question is like, where to from here? What, what role could mega studies play in the future? And in my mind, I think there's a lot more to the common task approach. So common task exercises, they've catalysed some of the key moments in machine learning and artificial intelligence. And you see that that progress against certain benchmarks on the screen, like really amazing progress and tangible. So can we bring the mega study even close to the common task approach? So common task tournaments, they have a few features that mega studies don't. They typically create an open playing field by making the data set generally available. Anyone can enter. People could have multiple cracks. And mega studies, at least those published today, tend to have interventions from a fairly narrow group of behavioural science teams. And I don't see to date any the evidence that behavioural science teams are more skilled at developing messages and say, marketers, the behavioural scientists that couldn't predict which would be better after all. So the question like poses how can we open up and democratise who provides interventions? I actually saw earlier this year there was a call for interventions from a group running, going to be running a happiness mega study, interventions to boost happiness, which is a great step, but I'm not sure if it ended up on the desk of any marketing agencies. And I really hope they recruit some weirdos. Now, most common task frameworks also allow each route of exploration in progress so teams can access the data outside tournaments to get the return to the problem again and again. Whereas mega studies to date are a one shot game. So could we have mega studies that run again and again, return to the gym chain and vaccination provider every year to get open entries and a process to whittle them down to the required number? Include a bunch of interventions from the previous year and, and really see if we can take move something forward like sometimes some of the limitations, the open task framework will become apparent. If we do that, if you run the same thing over and over again, you may tend to overfit. So you'll end up with something that's very good at maximising in your particular framework, say maximising attendance at a particular gym chain, but it may not generalise because you become very specific, capitalising on idiosyncrasies of that gym chain. And there may also be the marginal gains. So again, a lot of the around common task frameworks and machine learning have sort of led to a bit of a bit of a cap and they've plateaued. But I think there's something to be said for a process where we build on what we've learned rather than simply trial, scale and publish.
So taking inspiration for mega studies, the common task framework more seriously could really contribute to this. And of course, when you look at those performance curves on the screen, they're amazing. In the space of a decade, many tasks went from impossible for machines to machines being vastly superior to humans. And that's despite predictions that those advances of decades away. And I really hope that we can see a similar curve in understanding for the drivers of human behaviour. That would be an amazing thing. And that's where I wind up for today.
Susan Calvert
Wow, thank you so much, Jason. I feel like you've just raised the bar a bit. And we're going to need AI for these mega studies. I can see that 50 interventions and 60,000 plus participants are slightly terrifying. Thank you to all our presenters today, such excellent examples of innovation. So good to look what our future looks like. Very exciting. We're going to so jump now into questions and answers.
And our first question is for you, Bowen. We've had quite a few questions from the audience on the accuracy of your simulated eye tracking technology. I think we're all thinking of the productivity gains and the cost savings that are that your tech can deliver. So have you tested your algorithm against the eye tracking of real people?
Dr Bowen Fung
Yeah, that's a great question, Susan. And I'm going to pop up just an additional slide I've got here, which is going to be a little bit more impactful. But I guess the short answer is that we haven't tested it, but it's based on an open source algorithm that has been developed by a bunch of academics. And I'll thank Jason here for introducing the idea of the kind of common study or common task framework already, because this is something that's routinely used when it comes to kind of machine vision. And there are a number of different challenges that these academics will routinely kind of submit their algorithms to in order to benchmark them against real human progress. So typically what happens along certain metrics is you'll see these algorithms performing at around 88% accuracy compared to kind of real world examples. And I guess just to back up as well, I think that one of the important things to think about here is that the tool that we use is relatively limited almost by design to just work on static images. So it means that when you're looking at the images, when you're looking at these heat maps that have generated that, that are showing where attention is likely to fall, you've got your own internal model of what should be attracting attention as well. And so you can say, yes, this looks sensible to me. I think this is going to work relatively well. Or you can say, actually, wait a second, there's something off here, something's gone wrong. The machine's picking up something that's strong attention in a particular way. So doing due diligence like that and just making sure that it's working works very easily in the scenario that we're talking about here with the simulated eye tracking. It's also just interesting to delve into a just a tiny bit more detail about how the model works. It kind of does have a structure that looks a little bit like the visual human, the visual the human visual system. And it's been trained on a whole bunch of data that's been generated by real people as well. So it is matched up to being able to kind of try and pick out objects in the environment that are attractive to humans. And you see that because in the example I showed earlier with the energy bill, you see that the attention is kind of drawn to that dollar sign. The training data set doesn't actually explicitly have text. So text isn't something that the algorithm is paying attention to. It's only paying attention to those higher order characteristics of that text, like the meaning that that come from certain symbols like that, which is super fascinating.
Susan Calvert
Thank you so much, Bowen, that's really encouraging. I have a next question for Melissa. What challenges have you come across when working with behavioural systems mapping?
Melissa Hatty
It's actually overlaps with one of the questions that I've seen in the chat before, in terms of the behaviours and interactions that should be considered within context, particularly the kind of institutional and cultural context. And I guess one of the challenges we've come across is, the people that are in the room developing, identifying the behaviours and drawing the links between the behaviours. The map that we end up with is largely dependent on who those people are and what their experiences are. So in the context of the cyber security work that we did, we had a very diverse group of people from this set of organisations. But we had to recognise that their experiences of the problem state in their own organisations were very much unique to those people. And so being able to generalise to other organisations, potentially not so much. So I guess that's probably one of the main challenges. Thanks, Susan.
Susan Calvert
Thanks so much, Melissa and over to you, Jason. Mega studies, how quickly should BETA and other behavioural science teams around government and outside of government be adding these to our tool kits and what's it going to take to do that?
Dr Jason Collins
I think yes, I think that's a really important question to think about, Susan. But, and, and you know, ultimately the answer isn't going to be one where, yes, let's do mega studies and let's go out and do a mega study. But it's probably going to be reflected in the, the approach that behavioural teams take to some of the problems they have. So quite a typical process when you're doing, I suppose, a behavioural project, you've got a problem, you look at the behaviours maybe causing it, you try and diagnose and put some scientific framework around it, develop a bunch of interventions based on that, whittle them down based on your professional expertise, and then run a small experiment. And I think the thing about mega studies really shows to me is that at that point where you winnow down to a small number of interventions to experiment with at the end, there's actually a lot of value, perhaps sometimes going actually we've got a lot of people here, we're big, we're a big bank, we've got millions of customers, we communicate millions of times every day. We're a government agency and we send out 2,000,000 text messages a week, whatever it might be. And at that point going actually, maybe we should actually keep the funnel fairly wide at that point, let's run a really broad set of interventions, give them all a go. Because I think one of the things that's come through these mega studies is that we're not very good at predicting what's going to be most effective intervention beforehand. I think a little of that winnowing down, that trimming down we do before experimentation is probably getting rid of possibly some effective interventions. So actually being willing to run the experiments with more interventions could be a useful thing to do. Obviously like that, that trade off of power has to be thought through really carefully thinking about how to interpret experiments with many interventions. I didn't talk about it much today, but actually you'll be really careful about how you look at the winner. And, and because of course, sometimes winners in big experiments are there because of luck, or at least their effect size is exaggerated. But still, if you do that, think about that properly, use good statistical tools and, and, and make sure you've got make sure the sample matches, you could really learn something that's useful. The last thing to think about though, with mega studies too, is also how you deal with them over time. So one of my favourite sort of ever publications in the behavioural science space is still the old Test, Learn, Adapt that came out the behavioural insights team right at the beginning . And, and then one of the main things about that at the end is that adapt part. And when you run experiments, have like a big, big, you know, get a get a result, But it's like, it's not just going, there's my mega study, I'm done, It's actually doing it again and again. And so places like government departments, again, banks, like I imagine there's a lot of places where lots of communications going out and you can actually be doing this as an iterative process over time. It's not just a one off mega study, it's actually a series of mega studies where you're really learning, iterating, adapting. Thanks, Susan.
Susan Calvert
That's great advice. Thank you so much, Jason. We do in BETA help inform policy solutions by testing a range of interventions before they roll out. But we think 5 interventions is a lot. So, so we, we have a little bit of a gap there to fill. So we look forward to working with you on that.
The next question we have is back to you Bowen, and how do you handle generative AI hallucinations? So as we all know, AI chat bots have sometimes been shown to be wrong in a very confident manner. I suppose humans are sometimes too. But even if getting their inputs from good peer reviewed sources, how do you deal with it?
Dr Bowen Fung
The great, great, great question, Susan. I guess in the case of simulated eye tracking, like I said, it's a it's a little bit easier because you've just got the data there. If it's always named, you're going to instantly realise that there's something wrong with the case with PersonifAI, the persona generation and I guess other kind of large language models in general, this is a little bit more tricky. So there are kind of technical solutions to this problem. So the, the way that we've kind of built this, this custom LLM is to try and constrain the sources that it pulls from. And there are other techniques like retrieval, augmented generation, which can really, really, really narrow down and constrain these LLMs from the sources that they pull from. Having said that, all they really do is kind of reduce the risk that they start to hallucinate or they kind of make things up that aren't from verifiable sources. So you can never eliminate this risk completely. I'm, I'm essentially of the opinion and we try and make sure that the protocols kind of, within at least in BIT, are really strong to this effect, that part of the current responsible use of AI is essentially to kind of do a lot of due diligence to screen and to check outputs and to validate them. And we'll essentially never use results from these AI tools wholesale without kind of double checking all of these sources. I think it's really important to emphasise that these are tools that are here to kind of aid behavioural scientists. And behavioural scientists themselves should already be trained up to know how to, you know, do the ‘manual versions’, if this makes sense. That will help them use the tools more effectively, but it will also help to catch issues like hallucinations in the future. I think that's a big world. It's a, it's a kind of a bit of an evergreen problem for a lot of people that are kind of relying on AI tools at the moment is not to become over dependent on them. So it's something that I think we do have to pay attention to going into the future. Thanks, Susan.
Susan Calvert
Absolutely, thank you, Bowen.
The next question we've got for Jennifer and thank you so much to all our audience for asking such excellent questions. When would you not use behavioural systems mapping?
Jennifer Macklin
Thanks Susan. It's a really interesting question. I am really enamoured of behavioural system mapping. We're actually developing a course to be able to teach how to use it at each of these different steps in quite detail. But when I talk to some of our partners about it, I say it's even useful if you just have a piece of paper and you just, you know, draw a few things down to get some of your thoughts out. I think if you're tasked with a really narrow kind of task, like for example, how can you improve a letter, you know, to get people to, you know, pay a fine or sign up to something or, you know, go and do a particular task. Perhaps then say system mapping is not going to help pick the best message, you know, for the particular letter that you're doing. But it's interesting and Jason talks about mega studies that often those kind of really quantitative meta-analysis can leave us with questions that they often can't produce like really clear answers because there's a bit of uncertainty. And so we find that things like behavioural system mapping can actually introduce sort of a qualitative sort of decision making process to actually help. You know, if you look at all of those interventions in there and we're not really good at predicting which ones are likely to work, but behavioural system mapping can help predict which ones are less likely to work by actually understand which parts of the system, which constraints are not going to be addressed by the particular intervention. So I think it can actually be really helpful at any point of any project if you're doing a particular thing and you're not getting the outcome that you want. So if you're doing something and you're implementing interventions and you're getting the improvements that you want to see, that's great. But if you're not, or if it's not at the scale you want, what we found is that usually means that there's unidentified system constraints going on. And so actually taking that time to map out the behaviours, the interactions between those behaviours. And as I said, building in the actual barriers into the map as well, which can, for example, take account of things like, you know, institutional context. But what's really great about it is that it allows you to document those problems and those barriers to see where they rely on the behaviour out of other people. And so it can really help them choose where you want to focus. Do you want to focus with your ultimate target audience or do you want to do that? So I think that the answer is anytime you're not quite satisfied with the results that you're getting from your work, behavioural system, I think can help in some way.
Susan Calvert
That's fantastic. Thanks so much, Jennifer. And we're really excited to be using it in BETA.
Jason, for you. We're interested to hear your thoughts on the pros and cons of a mega study versus systematic review.
Dr Jason Collins
I have to admit, I quite like a good systematic review. It’s a super valuable input. But ultimately this comes back to this question of context that I talked a bit about before. So the great thing about a mega study is that you're putting in a single, single context. You've got a whole range of interventions that you're placing in that context and then you're able to get really good comparability within it. Like there's a really tough question about how much you can generalise it, but you're really hopefully going to nail that within context question. The systematic review, just by the nature of where it's likely pulling insight from is going to have much more cross context, your robustness. That's naturally what I'd expect there. But of but of course then when I'm there say, say I'm a gym provider or a government department or whatever it may be trying to implement something. I'd I guess generally in most, most reviews, there's enough uncertainty there, but little there quite often to actually go OK, well, of the various options that are that are here, how do I how do I make the final call? So, definitely both have their place, but ultimately, as you know, as I say, if you happen to have something that really closely matches the mega study context, you sort of go, maybe this is a little bit more valuable. If you're if you're sort of, you know, new context, a new problem, or perhaps even just thinking how robust is this going to be over time over with changes and the like, the systematic review might give you a little bit more insight. Cheers, Susan.
Susan Calvert
Absolutely. Thank you so much Jason.
And lucky last question, Bowen, have you tested PersonifAI against qualitative research based generation of personas and how similar are those outputs?
Dr Bowen Fung
Hey, Susan, we haven't actually successfully managed to do this yet. So we're looking for good opportunities where we think that we can have a kind of reliable qualitatively generated group of personas that we can kind of validate the persona, the automatically AI generated ones against. One of the difficulties I think with this is just the nature of personas, which is that they're not particularly objectively grounded in the first place, even though you can develop them and they can be accurate in terms of the qualitative insights that you gather from a population. They're always meant to be this kind of high level, almost stereotypes of individuals anyway. And so what we're trying to come up with is, is a bit more of a process where we can actually say, OK, well, yes, this is working in a way that is validated rather than just saying, OK, well, the AI generated ones look like this and then the kind of typical ethnographic approach ones look like this. How can we make that correspondence match? So this is something that we're definitely looking to do. And I think it relates back to the answer to the previous question, which is that we do kind of always take these outputs with a grain of salt. And when we are using the AI generated personas, we typically just use them for kind of early insights to kind of shape our thinking and to shape what might be possible in terms of intervention design. And we very, very rarely go ahead just relying on those and assuming that those are the kind of correct ones without then going and doing some more kind of qualitative research. Thanks, Susan.
Susan Calvert
Thanks so much, Bowen, and thank you so much to all of our presenters today. Bowen, Jennifer, Melissa and Jason, that has been absolutely fantastic. And thanks also to our audience for joining us and for your excellent questions. So you know, a recording of today's session will be on our website shortly. And as good BI practitioners, we'll send you out a survey later today to get your feedback on the session and, and so that we continually improve.
Our next session will be on the Wednesday the 20th of November and the topic will be on financial well-being. We're very pleased to be hearing from our own Doctor Bethany Jones from BETA and also Professor Michael Hiscox from Harvard University about some banking studies that he's been working on and also Doctor Iseult Cremen from the NSW Behavioural Insights Unit.
So we look forward to seeing you all. Thank you so much. Bye bye.
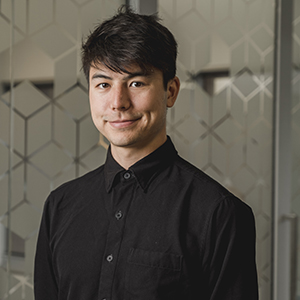
Dr Bowen Fung
Bowen is a Senior Research Advisor at The Behavioural Insights Team. Based out of Melbourne, Bowen has worked on projects in a diverse set of fields, including adolescent financial literacy, industrial policy, gambling, transport, infant medication, consumer protection, digital health, and workplace health and safety. Bowen has been involved in the development of a number of machine learning tools that are widely used across BIT.
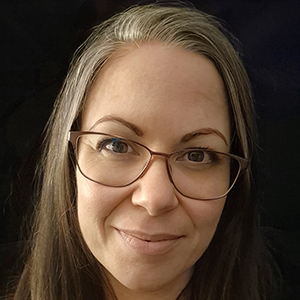
Jennifer Macklin (Downes)
Jennifer Macklin (Downes) is a Senior Research Fellow at BehaviourWorks Australia, with 15 years’ experience in applied behaviour change and social research to develop effective policies and programs for household, organisational and system-wide change towards more circular futures.
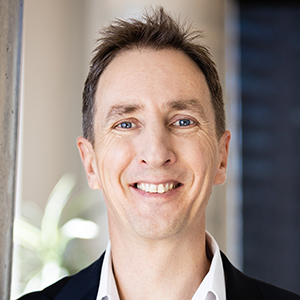
Dr Jason Collins
Jason is a Senior Lecturer at University of Technology Sydney (UTS) and Program Director for UTS’s Graduate Certificate and Master of Behavioural Economics. Previously, Jason co-founded and led PwC Australia’s behavioural economics practice, and built and led data science and consumer insights teams at the Australian Securities and Investments Commission (ASIC). Jason has a PhD from the University of Western Australia, researching the intersection of economics and evolutionary biology.
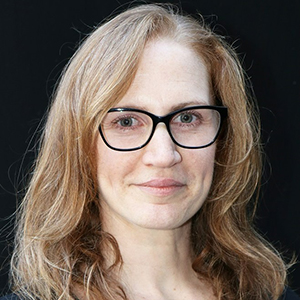
Melissa Hatty
Melissa Hatty is a Research Fellow at BehaviourWorks Australia, working across the environment, health & social, and education portfolios. Her research strengths are in survey design and quantitative data collection, database management and analyses, as well as qualitative and mixed methods approaches. Prior to joining BehaviourWorks Australia, Melissa worked in research and psychology roles across government, academia, healthcare, and private industry.