Transcript
Host
Thanks, everyone, for joining us today and welcome to the second session of BETA's BI Connect Virtual Series. Here we're exploring leading work across the behavioural insights industry. My name is Andrea Willis. I'm BETA's acting Managing Director. Thanks for joining us. But before we get started on behalf of all of the presenters joining me today, I'd like to acknowledge the Ngunnawal people as Traditional Custodians of the ACT and recognise other people or families with connection to the lands of the ACT and region. We acknowledge and respect their continuing culture and the contribution they make to the life of this city and this region. I would also like to acknowledge and welcome other Aboriginal and Torres Strait Islander people who may be attending today's event.
For those of us, those of you joining us for the first time and as a very brief introduction, BETA sits within the Department of the Prime Minister and Cabinet. We work across government and we apply behavioural insights to a range of different policies and programs. Our mission is to improve the lives of Australians by generating and applying evidence from the behavioural and social sciences to find solutions to complex policy problems. A core part of our mission involves building capability and has been a core part of our mission since we were established in. This event is one of many initiatives that we run to share knowledge and build awareness of behavioural insights and the role that it can have in supporting the development of government services, policies and programs. If you're feeling inspired by the end of today's presentation, please visit our website where you can find further information about BETA's projects as well as various tools and resources that can help you learn more about applying behavioural insights to a project of your own.
Today's session focuses on how behavioural insights are being applied to create safe and inclusive workplaces and importantly, identify areas for improvement. We have three presenters joining us here today and each will have around minutes to present their work. At the end of all three presentations we'll bring all the presenters back together for a panel-style Q&A. If you have any questions while the presentations are in progress though, please submit these via the Q&A function. You don't need to wait until the end. Our first speaker today is Dr. Liz Convery from the Behavioural Insights Team here in Australia. Liz is the principal advisor and the head of research in BIT's Australian Office. In addition to leading Australia's research function, she also leads major programmes of work in the areas of health care and work and safety. Liz holds a PhD in Health and Rehabilitation Sciences from the University of Queensland. Today Liz is going to be talking about the Australian gig economy, the nature of gig work, which is characterised by isolated, uncontrolled work environments and workers who are often more socioeconomically vulnerable than those who are traditionally employed. This necessitates a fresh look at the work health and safety strategies for these workers. Welcome, Liz. Thanks for joining us today. I'll pass over to you now.
Speaker 1
Thanks Andrea for the kind introduction and the invitation to speak today. It's great to be here with everybody. So I'm going to start off at a place where I think we all really, really know this. And that is the fact that what we do, how we behave, is not just the product of our intentions. Ground-breaking, right? Ground-breaking as Florals for spring. In simplest form, there is some truth to that. So we do need to have intentions in order to then act upon them. But of course, there's much more to that. So we all know that external factors, the environment and a whole host of cognitive biases also play a role in how we behave. So none of that is new. None of that is novel. But what I'm going to talk about today is the need to keep those things in mind when we want to understand work health and safety in the gig economy, which is a more novel application of those things. We also want to think about how we might use that knowledge to our advantage when we do the important work of designing interventions and developing policy to improve work health and safety in that space. So it's worth first briefly equating ourselves with the concept of work health and safety, or WHS as I'm going to call it from here on out. And then I'm going to talk a little bit more about what the gig economy actually is, what it consists of.
So starting with WHS, WHS focuses on the protection of the health, safety and welfare of people engaged in work. So very simple, but also very big, broad area. Here in Australia, employers are required by law to provide a safe working environment, and safety encompasses not just physical, but also psychosocial components. Work health and safety policy is set by Safe Work Australia. So at the federal level and then it's promoted and enforced at the state level by state regulators such as Safe Work New South Wales, Worksafe Victoria, etc. So WHS is traditionally kind of a dry topic, it makes people kind of tune out when it's raised in the workplace. But what makes it really interesting to those of us here today is that WHS isn't just about regulatory compliance. So it isn't just about going on to the Safe Work Australia website, downloading all their codes of practice and then just kind of ticking some boxes. It's also about individual decisions made by most managers and workers. It's about social norms around safety that become established within a workplace, and this is often referred to as growing a safety culture in the work health and safety policy area. And it's also about the individual habits that workers form over time. So in those respects, I think what makes WHS really interesting is that it's quite behavioural in many ways.
Work health and safety policy has been largely developed with traditional employment structures in mind. So thinking about people who are employed permanently, full-time, working sort of Monday to Friday, too. So what makes WHS even more interesting is thinking about it in the context of the gig economy, which upends a lot of those assumptions. And when we start thinking about the kinds of challenges that it might pose to our traditional understanding of workplace safety. So the gig economy is basically the intersection of three things. Caveat here is there is, of course, no one agreed upon definition of the gig economy, what it actually consists of. But this is the definition that I'm just going to run with today. So the gig economy is essentially the provision of on demand services provided by independent contractors, mediated by digital platforms. In Australia, as well as globally, you typically see three main categories of gig work. So you've got your rideshare. So things like Uber and Didi and Ola, you've got your meal delivery, Uber Eats, DoorDash, etc. and then task-based services like Airtasker. So appetite for the services provided by gig workers has really grown. A study showed that over two thirds of Australians use gig platforms regularly to buy goods and obtain various services. And the sector, the number of Australians engaged in gig work has kept pace with that growing demand. So in there were, Australians who worked in the gig economy and as of, which is unfortunately the last year for which we still have reliable figures, that number has risen to, So there's been a tripling of the number of gig workers in just four years. And you can imagine following the pandemic in, that number has ticked up even further, given the number of retail and hospitality workers that have flocked to gig work during that time. So obviously, the growth of the gig economy has a range of really interesting social and economic impacts. But what I'm going to focus on today is what it means for WHS specifically. And I'm going to talk you through some of the work that BIT has done in this space in collaboration with the Centre for Work Health and Safety.
So they are the research arm of Safe Work New South Wales. We've done quite a bit of work with them, but today I'm going to focus on two projects in particular. So first of all, a completed project about food delivery workers, and then I'm going to touch on an ongoing one about in-home care workers. So specifically the subset of in-home care workers who are part of the gig economy rather than part of the traditional workforce. And fun fact, there are actually different platforms in Australia through which people seeking aged care and disability care in their home can find people willing to provide those services. So it's kind of like the Airtasker-ization of aged care and disability care. Very, very interesting and growing area. So when we started doing both of these projects, very little was known about these particular populations. So we did quite a bit of exploratory work to start with.
We began, of course with some traditional methodologies, looking at conducting interviews with different stakeholders to get different perspectives, doing an online survey and of course reviewing the literature. But we also used quite a few sort of more innovative and immersive methods to really understand what it was like to do this work and what kinds of safety challenges that they faced. So with the food delivery project, we went out and did some field observations. We went to busy restaurant strips, sort of parked ourselves there and observed what kinds of behaviours, particularly safe or unsafe behaviours food delivery workers were engaged in. We conducted what's called a service safari, so that's when you assume the persona of a member of your community of interest and you do what they do. So in the case of these projects, it involves signing up to the various platforms, going through on boarding and paying close attention to any sort of WHS training that was offered, or in many cases was not offered at all. And in the case of the food delivery platforms actually working some shifts so we could know exactly what it was like to do this work for ourselves.
And the final thing we did was some textual analyses of Facebook posts that were made by workers. So it's one thing to get workers' perspectives by interviewing them. You go in there with your list of questions and you've developed those questions yourself. So they're sort of prioritised according to what you know. But when you look at things that people post in Facebook groups that are devoted to this type of work, you really get the sense of what is top of mind for them when it comes to safety.
So as a result of this exploratory work, we actually found that even though these two groups don't really seem to be too similar on the face of it, we found that they have some really important commonalities and those illuminate some of the unique WHS challenges that gig workers face as a whole. They also encounter some very similar cognitive biases that we might be able to think a little bit later down the track about leveraging when we are coming to solution development and policy design.
So first of all, their work is very isolated. So overwhelmingly, food delivery workers and in-home care workers work alone. They don't have any immediate oversight from colleagues or managers, and most of them don't even have managers in the traditional sense. They're all independent contractors sort of out there all by themselves. And one challenge this poses is the fact that there's rarely, if ever, anyone physically present with them to help them mitigate risks and hazards. So, for example, we found that verbal abuse and aggression are unfortunately very prominent hazards for both food delivery workers and in-home care workers. And this can come from a variety of sources. So for food delivery workers, it's often restaurant staff or members of the community as they're sort of riding by. And for in-home care workers, it can often be even more confronting. So coming from the client they're actually delivering services to or from a family member or friend of the client who might be physically present while they are there in someone's home delivering those services. So in those cases, they really are on their own. There isn't really anyone else who can step in and defuse the situation or apply any sanctions. So there's also, relatedly, a distinct lack of social norms when it comes to safe work behaviour. The vast majority of people don't wake up in the morning and think, okay, how can I be unsafe today? They do want to do their job in a in a healthy and safe manner, but there can often be a bit of a gap between how do I apply that sort of general principle of okay, being safe, how do I actually enact that with my behaviour? So gig workers don't really get the opportunity to see others like them practising safety, enacting safe behaviour, so they can't really feel the normative pressure to do the same.
So obviously, social norms, you need the social aspect of that to reinforce those norms. Gig workers' work environments are also quite uncontrolled, so food delivery workers drive or ride between different locations every shift. So it's different every day. And in-home care workers will work, obviously, as I said, in people's homes so they don't have any sort of control over the hazards that already exist when they walk in the door. And this sort of brings me to one of the really interesting and I think kind of relatively under acknowledged aspects of the gig economy. So the idea that it's not just redefining our relationship to work, how we do work, how we procure work, but it's also making us really reconsider the environments in which we work actually takes place. What is a workplace in the gig economy?
So as I mentioned before, WHS policy has been developed with traditional employment structures in mind, and that includes the definition of a workplace. But how do you apply those policies when your workplace is not an office or a warehouse or a factory floor, or even the same physical location every day? What is it? What does it mean if your workplace is someone's home or out on the road? And even more importantly, you're sharing that space with other people for whom it's not their workplace. So you're in someone's house, you're working and you're in your workplace, but they're not they're in their home. What does what does that mean when what does WHS actually mean when the physical boundaries of the workspace are so fluid? How can you encourage safe behaviour through smart workplace design if you've got little to no control over your physical choice architecture? So these are some questions to ponder. I've spent a lot of time pondering those questions, they are very interesting questions to think about, and we will come back to those questions in a little while.
The final thing, gig workers tend to be vulnerable in other ways. So many people who do food delivery work or in-home care work do it because they lack opportunities for more stable, better paid work and as a result, their mental risk benefit calculation might look a little different to someone in a more stable form of employment. So, for example, if you get paid per delivery, you are going to be incentivised to do as many deliveries as you can in a short a time as you can. So what does that mean for your appetite for taking risks? So are you thinking, okay, I've just got to do as many of these as I possibly can, so I'm going to speed, I'm going to run red lights, I'm going to take that shortcut across the pedestrian only plaza to get where I need to go. Are you going to be more likely to do those things? I think the answer is probably yes. And how does that change the likelihood that you'll experience harm from a particular hazard if you are putting yourself in that hazards way a little more often? So as I mentioned before, our work with the in-home care workers is still ongoing, which is why I'm not really talking about it too much. There's only so much I can actually say about it, but our food delivery worker project is complete, and it's also enough in the past that we can track some really nice policy impact, which is of course the whole reason we do the work that we do.
So we produced two reports based on our exploratory research. So one of them was talking about WHS from the worker perspective, one from the platform perspective, and we released those reports into the wild at what was a very, very tumultuous time for the industry. So the reports came out at the same time that five food delivery workers were killed on Sydney and Melbourne roads within a two month period. And at the same time a number of State Government departments here in New South Wales were starting to launch some initiatives in this area. So I can't overstate how much food delivery work dominated the headlines during this time. Every day for weeks on end, there was one story at least on the front page of the Sydney Morning Herald or The Age, talking about what was going on with food delivery workers and platforms and all that sort of thing. So it was a, in a perverse way, it was a nice time to be doing this sort of work because it was it was top of the tree for everybody involved.
So as part of some of the government initiatives, our State Minister for Better Regulation and our State Minister for Transport and Roads established a food delivery riders' task force. So part of the task force involved six of the global platforms and one pizza delivery chain. And together they committed to an industry action plan that was focused on improving their delivery workers safety. And what was especially satisfying about this was that the majority of the actions listed in the plan were drawn directly from our work and our subsequent solution- design workshop that four of the platforms had attended. Doesn't get much better than that, does it? When your work actually has a concrete positive impact, but wait, it does actually get better. So the cherry on top is if you look back at the Safe Work incident reporting statistics, so with the caveat that these are thankfully very small numbers we're dealing with here because the bar for reporting to Safe Work is actually quite high, used to be a very significant injury or death to be included in the stats, there's been a steady downward trend in the incident rate since the taskforce and the Industry Action Plan were put into place.
So back to some of the solutions. So moving on from the exploratory work into thinking about concrete solutions we can develop. So one of the key things that we had to keep in mind and this is something that I flagged a bit earlier, is the fact that food delivery workers don't operate in a workplace with consistently defined physical boundaries. And that's the case not just from one worker to the next, but also for the same worker from one shift to the next. Every day is a little bit different. But what is consistent from worker to worker and from shift to shift is the digital space they operate, the app they use to pick up delivery jobs. So if we can't modify their physical choice architecture to encourage safer behaviour, well let's think about their digital choice architecture. So that's where we focused in.
So we identified quite a few issues in our exploratory work. So we ended up focusing on just one of them. And one of the key problems that we uncovered was that there was a mismatch between what platforms intend delivery times to be and how workers were interpreting them. So if you're a food delivery worker, you pick up a job on the app and somewhere on the app it'll say, okay, you're delivering this food to the customer by p.m.. And workers were very logically and understandably interpreting this as, okay, this is my hard and fast deadline. If I miss that deadline or miss enough of the deadlines then I'm going to be penalised for the platform and potentially deactivated, it's perfectly logical. When we spoke to the platforms, it turns out they actually intend those delivery times to be more of a suggestion or range. Obviously they don't want workers to deliver things several hours late, but it's not the hard and fast deadline that it seemed to be. So we thought let's target that for trying to reduce speed on the road and increase safer driving and riding behaviour. So we worked with one of the platforms and a focus group of food delivery workers to design a messaging campaign that was going to go out via the platform's in-app comms system. So these weren't sort of emails coming from a third party, these were things coming directly from the platform themselves.
So we wanted to look at how do we get them to slow down and sort of reinterpret what these delivery times actually were. So here's what the messages looked like. So we started by explaining what delivery times actually mean using plain language. We used a very simple illustrative graphic. Sometimes I look at this and I think maybe this is too simple, but we were very constrained by what the platform would allow us to do in a message. All messages were personalised. One of the messages highlighted the fact that the majority of the platform's workers prioritised safety over speed, and that is actually a stat that we drew from our exploratory survey that we did earlier in the project. So it's actually it is a true statistic that we're sharing. So basically trying to use digital means of messaging means to create that social norm of safety.
One of the other messages asked workers to make a plan now for what they were going to do when they were under the pump and really busy later. So they were seeing these messages at the beginning of their shift. And that's an important point as well. We made sure that the platform only sent the message to be seen when each worker logged on to begin their shift. So we didn't want any of these messages popping up while they were driving or riding while they were in the middle of a delivery, because obviously that would have the opposite impact to what we want. We don't want them any more distracted than they are.
So we tested the messages using a two armed RCT, very simple trial design. So we randomised workers to either the treatment group who got one message a week for four weeks or to the control group who got nothing. We had over, workers in our trial, half in each group and they all came from Sydney or Melbourne. And when we got our final data set, it really brought the message home to me how busy and prolific these platforms really are. So in just a month, in just Sydney and Melbourne, our dataset included million deliveries. So you can see just how well entrenched one platform is in in the way we sort of live our lives is really interesting.
Okay, so what did we find? Well, we found that the messages had no effect. Bummer. I was I was just so gutted by this when we did the analysis. However, what we did uncover during the trial, I think, was arguably even more interesting. And this is not just me trying to make myself feel better about getting a null result. So we delved a little bit deeper into the data and we found that only about 17% of the workers in the treatment group even opened the messages. So of course they had no effect. You can't have a message having an effect on someone if they're not even reading it in the first place. So we decided, let's do a treatment on the treated analysis. Look only at the workers who did read the messages and lo and behold, they actually were effective. They recorded travel speeds that were about 3% slower than the control group, which with this side end was a significant difference, even though in real terms it only amounts to about half a kilometre an hour slower travel speed.
So what does all this tell us? And this is why I'm just going to step up on my research soapbox for just a moment, if you'll indulge me, that regardless of the outcome of a trial, whether it's what you're hoping to see or whether it's a null result, I always think it's really important to look for the lessons. To look for what can I take from this? What can I learn from this to then apply in subsequent research or another project? So three things I think we learned from this trial. So first of all, that the messages actually are effective. But second, and I think this is probably the most important lesson, that worker engagement is a problem that absolutely must be tackled first. It's a much more upstream problem and it is sort of the gateway to everything else. There is no way you can get people to have any sort of widespread effect of any sort of behavioural strategy if they're not engaged in the first place. And the third thing of course, is that it's important to test something before you go ahead and implement it. And I know those of you who are familiar with BIT and our ethos, we do bang on about trials and evaluations and how important it is to test something. I think this is a perfect example of why and the importance of testing. So you can imagine in an alternative universe that the platform had come to us purely on a consulting basis and said, look can you give us some advice on how to get our workers to slow down to be safer? And we said, well, based on our knowledge of behavioural science, here's a suite of messages that should work. And then they just went ahead and rolled it out and scaled it across their entire fleet. Turns out the messages don't actually work, so if they had done that, they would have been spending time, money, effort, resources for no effect and possibly even some sort of backfire, peppering their fleet with messages, just creating noise for no good reason at all, maybe even distracting them from receiving a more important message that actually did work.
So it's clear that worker engagement is the problem to be tackled. And our hypothesis is actually that this is also likely to be a challenge among in-home care workers and other gig workers as well. So why is that? So here are just a few things we think are likely to be at play for gig workers in general. So first and foremost, cognitive overload, food delivery work and home care work are extraordinarily demanding jobs, safety messaging that isn't immediately and critically relevant to the task they're doing is likely to be just dismissed, ignored, overlooked, whatever. They're not going to be paying attention to that because it's not directly relevant to what's in front of them. Gig work is unstable. It's inconsistent. Workers are much more likely because of those things to be more focused on their immediate income or delivering good customer service to the customer in front of them, than thinking about longer term issues like health and safety. So some of the more high profile ways that you can be harmed at work are sort of things that have a very immediate impact, like being hit by a car, for example. But most work health and safety hazards, you're sort of in it for the long run. So if you take the example I gave at the beginning about verbal abuse and harassment, maybe one or two things, you're able to just kind of let roll off you. But a steady drumbeat of that kind of thing over time is really going to have some fairly significant consequences or, you know, even thinking about just very typical sort of musculoskeletal injuries, you don't really incur an injury necessarily from one time. It's over many, many years of doing the same kind of repetitive movement that all of a sudden you realise that you've incurred a permanent injury. So if you are thinking about, you know, what's in front of you, maximising income, satisfying the customer you have in front of you, you are not necessarily going to have the headspace for thinking about anything longer term.
And finally, relating to what I spoke about earlier about uncontrolled workspaces. So if you have little to no control over the hazards that are present in your workspace, you may very logically and sensibly conclude that anything you try to do to try to tip the balance to try to improve your safety really just won't have much of an effect. So there are no doubt many other factors that contribute to this, and I'm sure that many are sort of popping up in your mind as you as you listen to me go through this list. But these are just a few to think about.
And I think on that note, I will leave it there and thank everyone for their time and attention and pass back to Andrea. Thanks, please.
Host
That was really interesting. And I loved your message about drawing lessons and meaning from unexpected or surprising results. That's something that's really important here. Better as well. Before we move on to the next presentation, I just wanted to remind participants, please leave any questions that you have as you have them in the Q&A chat and we'll come back to them at the end of the session.
Well, now move on to our next speaker, Dr. David Smearon. David is a lecturer at the University of Queensland School of Economics, and his research focuses on where behavioural economics can be applied to social policy. David has a Ph.D. from the Tinbergen Institute and the University of Amsterdam and previously worked as a PODER, which I had to look up, which is policy design and evaluation research in developing countries, which is quite a mouthful. So I can see why they came up with an acronym. So he's A PODER Fellow at Bocconi University in Milan. Prior to his academic career, David spent three years working for the Australian Department of Treasury as a policy analyst. And if you're not already suitably impressed by his professional and academic background, David is also a chess grandmaster. Today, David is presenting a field experiment on gig workers discrimination against gay job posters. I'll pass over to you now, David. Thanks.
Speaker 2
Thanks. Thanks a lot for the nice introduction. So I'm currently working as a behavioural economist at the University of Queensland. I work on a lot of interdisciplinary projects, some field projects, some work in developing countries and yes, even some chess research as well. Where I particularly look at gender differences across countries in chess. But today I'm looking at something quite different to those topics, but much related to what Liz just spoke about. So it's a very nice transition. This project is about discrimination in the gig economy on the dimension of sexual orientation. So I'll provide a little motivation. Also provide a bit of background on discrimination. More generally and particularly how it's approached by researchers.
For some of you, this will be familiar material. For others, I think it's quite insightful to see how research has really come a long way when it comes to identifying discrimination. First, a definition economists. We love definitions. Discrimination is one of those things. We kind of all know what it is. But just to put it a bit more formally in economic terms, it's a situation where members of a minority group are treated differentially typically less favourably. The members of the majority group with otherwise identical characteristics in similar circumstance. And it's that last part which is kind of particular relevance to traditional economists, because when we think about the labour market and efficiencies, any sort of discrimination that fits this definition is going to lead to inefficiencies. And that's why it matters for the economy as well as its social and ethical implications. Now how to measure it. We can't just go around asking people, do you discriminate? That's typically not going to be very reliable material. But since the early 2000s, the ‘correspondence study’ has become the gold standard for methodology in measuring discrimination. Let me explain to you the general set up of how it works.
What the research will do is typically prepare thousands of job applications or CV's and send them out to real jobs that are being posted around the place. What they'll do is they'll create a pair of applications each time, and those applications or CV's will be identical in all respects, except for just the dimension on which you want to investigate discrimination. So think about gender, for example. You just make it either a male applicant or a woman applicant. Now, these are fictitious applicants, which means that we can keep everything else identical. What the research will do then is measure the call back rate. So how often that CV gets a call back to interview at the first stage of hiring? And if you see a difference between the group of CVS with, say, male names and the group of CVS with female names, we can identify that as discrimination. So it's very simple. It's very clean in terms of identifying discrimination and it's very popular.
There have been hundreds of correspondence studies since the early s and still going on today across all dimensions of discrimination that you could possibly imagine and the ease of which the correspondence study can be implemented and the I guess the cleanness or cleanliness should be cleanness of the identification combined with a famous study about orchestra auditions, has really made this a very popular area for research. So the orchestra study you may have heard about before because it was conducted in , but made its way into, I guess, the public awareness in terms of podcasts and what I like to call airport books that you can buy as well. The airport study was conducted in the US where in the first situation men and women would play their instrument at an audition for an orchestra. In the second situation, there would be a curtain put up so that the judges wouldn't know the gender of the person playing the instrument. And the famous result is that these blind auditions increase women's chances of being selected in orchestras by 50%, a huge margin. So since then, and with all the implications that have come from correspondence studies, the policy implications seem to be very clear.
Anonymous your CVs, anonymized job applications, you reduce the bias we in behavioural insights, we love these low hanging fruit, these small nudges that can have big effects, and it's been used by behavioural insight teams around the world and also spin offs in the industry such as the applied company that was started by some people who worked for this in the UK. You can probably imagine there's a there's a however coming up and that's, that's the next slide however. So blind auditions that are an organisation several criticisms have come to light in recent years and actually some even earlier that didn't get the same publicity as the famous orchestra study that actually went back and analysed the original data and found there was actually no evidence at all that women did better in blind auditions. And this was kind of quite shocking. And it's not that well known. I'm also quite guilty of using the orchestra study in my lectures as well.
Furthermore, there's been little hard evidence that anonymizing surveys and job applications have that much of an impact in reducing hiring discrimination. It's something good the beta team tried as well and going to what Liz was saying before, I think it's incredibly important that the BETA team did run this experiment and tried it rather than just rolling out a policy. They tried blind blinding CVs and found that it actually decreased the chances of female and ethnic minority hiring in the Australian Public Service. And in the final report that was released had a very important comment, which is we should perhaps be looking for discrimination in other stages of hiring besides the initial review stage. And I think that's a big motivation for our project as well.
So what are the drawbacks of this correspondence methodology? Well, there are three big ones. The first, which is kind of highlighted a little bit by BETA’s comment in the report is that discrimination at the call back stage age is not hiring outcomes. It's maybe measuring a different sort of discrimination, but even worse than that, it could actually be confounding or hiding true discrimination. Why might that be the case? Well, at the initial hiring stage, there are corporate and social pressures to actually increase the diversity of your quota because there are low stage, low stakes. You're just calling people in for that very first interview. So your corporate image concerns can actually outweigh any biases that might exist within the organisation when it comes to hiring.
The second thing is this very clean, simple methodology is great at identifying an effect between two groups, but doesn't tell us why. It gives us no contextual guidance as to the sort of environmental factors that might affect whether people are more likely to discriminate or not, or more likely to be discriminated against. And in that sense, it doesn't tell us whether the discrimination is taste based, which is the way in typical parlance we talk about discrimination or bias or statistical. Now, this is an economics term, statistical discrimination. But what it means is a kind of rational response to a low information or high uncertainty environment. So in the blind orchestra audition example, for instance, men still performed better than women on average in the blind conditions. And if a judge were to know that on average in the sample, men seemed to perform better than women and they don't place a lot of stock in just one audition, they might take the person's gender as an imperfect signal to extrapolate that future productivity, which can consist, which can also exist in other sort of workplace hiring environments as well. Now, why does this matter? Well, academics like to care about these sort of mechanisms, but from a policy perspective, it's even more important if the root cause of discrimination is statistical discrimination. The policy response is reduce the uncertainty in your hiring situation and increase the availability of information or from a behavioural perspective, maybe increase the salience of certain types of information. But if it's a taste based discrimination, it's quite different. The policy response might sort of centre around workplace initiatives and programs around deep bias. So these are very different sort of things.
The third thing that I'll mention is perhaps why we've seen so many correspondence studies that have focussed on gender or ethnicity and not on other forms of discrimination or other dimensions, such as sexual, and that is in the correspondence study It's very easy to send a pure signal of gender. You have a male applicant, you have a female applicant, but it's very difficult to do that for, say, sexual orientation. Now, one way you might think to do it is to put on your CV. I was the president of my university's LGBT organisation, but that doesn't necessarily signal your sexual orientation. And even if it is correlated, it might signal other things such as you're an activist or you've got left leaning personality traits. So you're not necessarily measuring sexual orientation discrimination in the final wash up as well. So that might explain why there hasn't been as much research on this particular dimension. But there are other reasons why we should be interested in sexual orientation discrimination.
Even in Australia, a country which I think we can all agree parts ourselves on the back of a fair bit when it comes to equality upon this dimension. However, we're just Australia globally as opposed to, say, gender or race. Most countries don't have explicit laws against sexual orientation discrimination in the workplace and even among those countries that do quite often, we find that LGBT workers have significantly higher rates of poverty and unemployment and lower wages, which we all find to some extent also in Australia. Now, in the US, one in four LGBT workers have reported being fired or not being hired specifically because of their identity. Obviously, those are self-reported results, but still a very high percentage. And even after the US Supreme Court passed a ruling to extend the civil rights protections to sexual orientation, in the months following that ruling, one in ten LGBT workers reported a hiring or firing discriminatory experience. What about in Australia? Obviously we're better than the US where we are in terms of rankings. We're actually ranked the in the world for LGBT legal protections and in a separate study, for social acceptance. And Melbourne is currently ranked fourth in terms of the queer friendly cities around the world. Most of us know in same sex marriage was legalised in Australia after about two thirds of the country voted yes In a postal survey. And there's been one study that showed that in the years from around to, there's been close to average wage convergence amongst gay and straight men in Australia. So all really good news.
A couple of question marks, though. Most of the data that we have for these rankings are again based from self-reported surveys. LGBT workers have twice the rate of unemployment still in Australia. Organisations rarely provide clear rationales for their selection, so it's very hard to distinguish whether any sort of hiring or firing behaviour has happened because of sexual orientation or genuine qualification differences. And perhaps most importantly, there have been criticisms of pink washing. Pink washing, kind of like greenwashing in an environmental context. Is this idea that in corporate and social environments, image concerns can actually lead to, for example, increasing the diversity of your initial interviewing stages and putting other sorts of initiatives in place to present a queer friendly image which may be actually masking hidden day-to-day discrimination. So we decided to conduct our study in Australia, a very queer, friendly, friendly place, so a place which we thought would actually be quite to find discrimination.
So ask three questions. The first one is the LGBT plus discrimination in the Australian economy. Second, if there is discrimination, is it affected by physical proximity between gig economy workers and users? And third, does the likelihood of discrimination differ across cities, genders and local LGBT plus attitudes? Why do we choose these particular environments? Well, let's go through the gig economy. I was going to talk about how important it is as a growing industry, but this is already covered that. So hopefully by now you're convinced that it's a really important and growing market to focus on. But second, from an academic perspective, we're looking at worker discrimination towards use. So we're flipping the market, which means that there are higher stakes at play if a worker chooses to accept a job for from a profile that they might be a gay profile, they're essentially leaving money on the table immediately. So it's not like initial call-backs. This is real biting discrimination that actually hurts the person who engages in the bias. Second, we're looking at sexual orientation, not just because it's under study or not, just because LGBT workers are affected amongst a number of economic dimensions, but also because this particular dimension in this particular gig economy market removes the likelihood of statistical based discrimination, that is. And bias behaviour that can be rationalised in some form, which means we're really testing for, I guess kind of like that pure bias when we use the term discrimination in typical conversation. And finally, we're looking at physical proximity as a moderator because most correspondence studies, as I mentioned before, don't really tell us the why. They don't tell us the contextual factors underneath discrimination. And we didn't just choose this particular moderator just out of thin air. We chose it because it is theoretically motivated, although I won't go into that literature today and it's practically relevant. More and more companies are moving towards virtual based interactions with both users and other colleagues in the workplace. And in fact, one in ten new job postings mention remote work. So that's the motivation and that's set up.
Let's go to the experiment, as we call it. It's an RCT, but in economic terms we call it an experiment. We pre-registered the study, one of the largest online marketplaces in Australia, which as I mentioned before, it's a very queer, friendly country. I mentioned also that I pre-registered it just as an aside. Quite often coming from a policy background, I can well appreciate the frustration that behavioural insights teams must feel when they apply something for an academic study and find that it doesn’t work in the real world. I very much appreciate that and it's something that I'm quite passionate about. So if you ever encounter some research by an academic and you're a bit sceptical about whether to apply it or not, please ask them. If they preregistered this study, they probably won't like the question. Please ask them. Okay, so that's the setting.
Now, in this particular sort of platform, employers or taskers, if you want to call it, can post job ads for simple work tasks and I'm going to call them workers who can bid to perform those tasks for payment. And that could be odd jobs or jobs around the house, for instance, or services. We ran over job ads that we posted from fictitious male profiles across six Australian cities from March and July of , and we manipulated to treatment variables orthogonal, which turns it into what's known as a two times two design. So half of the job ads were posted by a fictitious gay male profile. Half of the job ads were posted by a straight male profile and then also we varied. Half were for jobs that were in what we considered to be close physical proximity, typically inside, inside jobs, inside the house where you need an extra set of hands. And half were outside. Typically something like yard work or cleaning up or weeding or something. Now, to manipulate sexual orientation, as I mentioned before, this has been somewhat controversial in this literature. Putting things like membership of organisations may signify things above and beyond sexual orientation. Here what we did is that we manipulated the profile photo that's used on the platform and also a reference to the partner’s sex as well.
So an example of how it looks, these are those four different treatment groups that we've got in total varying sexual orientation and proximity. And I've just highlighted sort of the differences going across horizontally, the differences in the tasks that you might see. So, for instance, looking at the bottom row, this is inside tasks. The first column, the straight task moving heavy furniture need a set of hands to help move the fridge. My wife will let you in and let you know where she wants to put it. In the second one, my husband and the differences will also be in the profile photos. There will be a heterosexual couple in the one on the left and there will be a gay couple on the right. So very small changes, but enough that it's salient. What happens on the platform when a job is posted is that workers were typically scrolling rapidly throughout the day through these different advertised tasks, can essentially do one of three things. The task will be there with an advertised price, say, for instance, $100 for hours work. You can click offer, which is essentially entering or willingness to enter a binding contract to perform that task for that price. You can make it counter offer which 95% of the time, means putting a higher price. So saying, yes, I will do it, but for $120, if that is then accepted by the tasker. A sorry, by that, by the by the employer, then that's a binding contract as well. Or you can make a comment which is a slightly weaker form of interaction. It signals a willingness to engage with the request, typically to ask more details about the particular job.
There are many outcome variables that we looked at because we weren't sure before running the experiment. What sort of things would be important? Also, what forms of any sort of discrimination might take. So what we did is we took those three actions that you saw before and took sort of combinations of them to come up with seven, seven separate outcome variables that are obviously correlated with each other, but might represent different forms of interaction. For instance, number seven, there is the counter offer amount that's put down, which might, for instance, signify some sort of gay penalty, which is different to not accepting an offer at all.
So there were seven main outcome variables and, also an eighth there that we were interested in for our robustness checks, which is the worker quality. So of all the workers who interact with a certain job on a five star rating, what sort of quality are you getting? Now, with many outcome variables, this again goes to the importance of pre-registration. We could have run these seven or eight outcome variables and then just chosen one or two that are significant. It's a terrible way to do research. So what we did is we put all of these down upfront with the idea being that we are looking for a general pattern of discrimination. So we would only really say that discrimination exists if we had at least half of these being statistically significant, but also hopefully it would give us some insights into what sort of form the discrimination takes.
Now when we go to the results, I'm first going to put a really big scary graph on the screen. The idea is that for people who are particularly data minded, they can look at that and pretty much get all the information that they want about the rest of the study and then kind of cruise through the rest of the talk. Those of you who don't like graphs at all, don't worry, we'll walk through it and then we'll get to the policy implications a little bit later on. So the big graph, which is I was always told when you do an academic paper, people only have to remember one figure maximum. So this is the one figure, but it's obviously a very complex figure. You probably have to squint a little bit. But what it is, all those black dots that are plotted there represent one test or one regression, all the dots down the bottom with the sort of blue circles and everything in the panels there that tells you what are the different things that went in to that particular regression. But as you can see, we did many, many tests represented by all of those black dots in the main panel there. Now, the zero represents no discrimination. They're all put on the same scale, which roughly speaking, equates to percentages. Not exactly, but close enough that you can use that as a rule of thumb. So a black dot that moves up the scale that is that is north of the zero represents, roughly speaking, a percentage level of discrimination against LGBT+ profiles in our experiment.
So you could already see that most of them are above zero. But what's most important here is actually down in the middle panel at the bottom here. So this middle panel or second to bottom panel represents whether the task for post inside the house or outside the house or all of them pooled together and this sort of line of black dots here where, the arrows pointing on the right represents the close proximity to us, the task inside the house. So if we just zero on those sort of regressions, we can see that those dots are, for the most part, significantly above the zero. And moving into that sort of 15 to 20% level of discrimination. So the overall pattern is saying, yeah, there's some evidence of general discrimination, but it's pretty much totally driven by tasks that are inside the house. Okay, so that's all the graph is saying in total. I'll go through the results step by step and also the breakdown of the results by heterogeneity as well. But the main result is that proximity matters a lot. So pooling all the proximity and just looking at the okay, this is straight profiles and how workers reacted to them. We see slight levels of discrimination, sometimes significant, sometimes not, not enough to be particularly worrisome, I would say, from a normative perspective.
But when we look just those inside tasks, the results are very strong. It's completely driven by those inside tasks. And we're looking at 15 to 20% discrimination on pretty much all outcome measures. In terms of the secondary results, we broke it up by male workers versus female workers and how they reacted to the gay versus straight profiles. Remember, we're only we only had male profiles in our experiment, so we had gay or straight male profiles. We actually found that female workers, while representing a smaller sample in our experiment, didn't exhibit any noticeable discrimination at all. It was all driven by male workers.
The final result is when we looked across the six cities in our experiment, we saw no substantial difference. They were all pretty much the same in the level of discrimination, except for Melbourne, the fourth most queer city in the world, a queer friendly city, I should say, exhibited by far the strongest and most significant level of discrimination, which was more than 20% on all the measures in our experiment. We have some hypotheses for that, but I won't go through it for time reasons. And this thought the last results mentioned here, what we did is we looked at the results by suburb, so our tasks were posted within a suburb in a city and we compared that to the voting patterns per local area in the same sex marriage postal survey, expecting that areas that voted yes to gay marriage with higher shares would exhibit lower levels of discrimination. We didn't find that at all. In fact, for the most part we found a weak but negative association. So in general, areas that voted a higher share of yes towards gay marriage exhibited higher discrimination in our experiment.
Final thing I mentioned before, the outcome variable about work equality. There's a particular theoretical or academic reason why we were interested in this. Remember, economists love to argue about is a taste based or statistical discrimination, and it's also of policy relevance as well in terms of how do you respond to discrimination. So an argument can always be made somehow that there's some rationalisation for the discrimination. For instance you might imagine that there's some unobserved reason unobserved to us why workers on the platform might prefer not to do jobs for gay requests. It might be that the more experience that you have in the task working on the platform, you come to appreciate these and observe statistical differences. So what that would is that more experienced workers should show more of a bias because they don't want to work for gay profiles. On the other hand, if its taste based, then we should actually imagine those with higher worker quality to incur a higher marginal cost for performing a task at the same price, which means that they are more likely to be biased towards engaging with straight jobs. So we'd expect more discrimination from higher quality workers if it's a taste based explanation. And indeed that's what we find. So that's the main reason for that analysis. But it also tells us that it's hard to think of any sort of rational explanation for why this bias is going on. So there's that scary graph again, but I won't talk about it just to sort of sum up the pattern of results again, to say that there's discrimination but only in close proximity.
And when we look at the outcome variables, the other pattern we see is that it's mainly related to engagement, whether or not you interact at all with the advertised task as opposed to saying, Yeah, I'll do it, but give me more money, which would be like a gay penalty or premium on the price. So we don't see that premium in the price. We just saw lower engagement. And that's actually consistent with one of the few papers that's in a sort of similar ballpark to what we do. This was done on Airbnb and it looked at the responses from hosts. When gay couples versus straight couples applied to stay at an Airbnb residence and they found that there was lower acceptance of gay male couples, but that this was again driven by just not responding to them at all. So this sort of silent discrimination, which is consistent with what we find as well. Okay.
Finally, the policy lessons for general policy lessons that we can take away from our particular study. The first remember, we chose a pretty difficult situation to find LGBT discrimination, choosing Australia of all places. And with Melbourne taking up a pretty big share of our sample. So even in progressive countries there can be this sort of hidden or silent discrimination below the surface and it might be really hard to imagine it, identify it, or even expect it in a country that is perhaps a little bit masked by so-called pink washing or image concerns, play a big role and are also unlikely to be captured by traditional correspondence studies. We've only looked at this in the gig economy when it comes to sexual orientation discrimination, but you can also think for yourselves how you would imagine similar levels of discrimination, perhaps more or perhaps less for other dimensions, which could be gender, but also things like Aboriginal or Torres Strait Islander status, age, disability and so forth.
The second, going back to what Liz was talking about in her presentation, discrimination is especially important in the gig. Economy is growing, it is massive. Everybody's using it here. We're looking at discrimination against users, which is a bigger share of the Australian population than the worker base. And because it is so quickly expanding, it's less regulated. People using it are slightly more vulnerable than traditional workspaces. So it can be a lot harder to identify silent discrimination. There have been some successes to date. With those successes, change has largely been driven by first academic research. Second, the publicity that's come from that.
And then third, that publicity sort of prompting the platforms to lead the response. To date, there has been little initiatives driven from the policy side of things. The third is kind of a little bit broader to the particular focus of our experiment, which is to say that physical proximity seems to matter for discrimination. And like I said earlier, we didn't just pull that out of the air. Physical proximity is pretty important in a climate where many more workplaces and industries have shifted towards virtual and digital interactions, and with the growth of remote work has become more and more prevalent. Now, on the one hand, with more and more remote work, you might say, well, our study shows a good thing. There's no discrimination when they're far away from each other. And that may be true. But on the other hand, it may be that more physical contact and social contact with people from groups that you don't normally associate with helps you to eliminate your own, perhaps unconscious biases. And that process is not going to be in place anymore. So what does that mean?
Well, it means that for industries and policymakers, they might have existing policies in place around training for unconscious bias, but it might be time to think about expanding that, to incorporate both remote work and virtual interaction settings with an explicit focus on trying to trying to highlight the effect of physical proximity on silent discrimination. Okay, that's all I've got for you. Thanks very much.
Host
Thanks so much, David. That was great. Really, really interesting study. And I loved the little lessons on the high quality, robust research design that we should all employing at work that was sprinkled through that presentation as we went. Thank you.
Allow move on to our final speaker today, which is BETA’s own Nick Hilderson. He's an advisor within BETA. And prior to joining us he worked at the Department of Employment in the Behavioural Economics. Mathematical Science and Economics, which you'll come to appreciate as he goes through the research project that he ran today.
Today, Nick is going to be presenting on some recent research that our team conducted using new methodology to understand gendered language in cybersecurity, job ads. In case you're wondering why such a niche focus for this research, job ads are obviously a key mechanism for employers to attract a diverse workforce, and cybersecurity is one of Australia's most gender skewed industries, with Women making up only 17% of the workforce. I won’t steal anymore of your thunder, Nick. So I'll pass over to you now.
Speaker 3
Cool, thank you, Andrea. So yeah, so I'll get started. So today of discuss the problem that cyber security facing and then we're going to answer sort of two questions. How do you write job ads in a way that attract more women? And what does the current state of Australia look like? So people are currently writing ads in a way that attracts women or are they not? Spoilers, they’re not.
So the problem. So cybersecurity is a really heavily male dominated occupation at the same time as having workforce shortages, which doesn't really make sense, they face acute work post shortages. So there's currently, job openings for cyber security roles across the country and over time, the number of workers to positions is only going to get worse. There's going to be more and more positions where they can't fill them. At the same time, cyber security is one of the most male dominated occupations in the country. As Andrea was saying 17% of the workforce is women and in some technical areas less than that. And we also found looking at ABS data that a lot of women who actually have relevant qualifications for cybersecurity are not working in that industry. So there's a mismatch between all the women that studied it. They're not working in I.T. so that they're either not applying for those jobs or they face some discrimination along the way that mean that they don't work in that industry. So how do you write job ads in a way that attracts women? So let's first try and understand, I guess, what a job ad is in terms of a signal.
The job, I guess, is one of the first way as an employer and a potential employee interact with one another a bit like, yeah, the tasking talk we were just listening to. So they help individuals decide whether or not to apply for a job. And the way you can understand the predictions of whether people intend to apply is that each person organisation fit the. Do you think you will fit into this workplace? Do you think you'll get along with your potential colleagues? And then the other part is the characteristics of the job itself. So am I qualified? I can't apply for an engineering job because I do not have an engineering degree. So the characteristics of the job itself mean that I would not be able to apply. So what we can think about is this is also an opportunity for employees, employers to potentially signal to potential employees, discriminate about whether or not they should apply for the job. So they it's an opportunity for them to essentially signal that they only want a particular type of worker and that in this case, this is probably we want this man. We want this man because we know we had a man in the past and this guy is great.
So the four ways that we looked at how job ads can be written in a way that I guess discourages women from applying is the first one is gendered language, which there's a decent amount of evidence for. So gendered language is the idea that you can focus on stereotypes in your job ads. And these are either more masculine stereotypes or more than the stereotypes. So for more masculine stereotypes, it's the idea that you have, like someone who likes to take charge, they're like a leader. They are really good at problem solving, sort of all stereotypes of men. The feminine stereotypes that the idea that you're caring, compassionate, you like a really good at looking after people's feelings and so what the evidence shows is that if you focus more on masculine stereotypes, your job, you'll have less women apply for those jobs. The next, I guess, is based on the research in cyber security might have issues with hiring women as the idea that cyber really focuses on the stereotype of we're all hackers in hoodies what is there even in the media they will post stuff It'll be some guy he'll have a hoodie on, he'll be typing on the computer, they'll be green text on his computer and so if you think that's what a lot of cyber security is, that's not what women there's not a woman up there who's doing that. And so women don't think they'll fit into the culture of this organisation. The other and they're sort of like the if you think about these, both of these are fit into organisation person fit. So it's like how will I fit into these workplaces?
The next two is flexibility and requirements, so flexibility, but talking about whether you have family friendly policies, good paid parental leave, flexible part time arrangements, job sharing. So there's pretty good evidence that more women will apply. If you have more, you offer flexible working conditions in a job. And you can think of this as women usually take up the burden of care in a lot of family relationships, and therefore they might need to access these flexible working conditions more than them and typically would. And the next thing is requirements. There's a saying that women apply for a job when they only make 90% of the requirements. It's actually no evidence of that statement. But there is some sort of weak evidence out there that lower qualified men will apply for a job at a higher rate than lower qualified women, but which is sort of mixed. I don't know if you really want to attract low qualified men anyway, but so we ended up looking at the number of requirements and jobs. And if they're really high, I guess the idea is that they have a lot more requirements then women won’t apply. So what does the current state of Australian jobs look at?
The first, I'm going to talk about how we I guess how do we how do you measure this? So we analysed most of ads in Australia, so we work with in collaboration with Jobs and Skills Australia who have access to a very fancy asset Lightcast, which basically has 60% of all job ads posted online from to. Well, it gets updated daily so all have to and when we conducted the analysis is around million job ads. So we in this analysis, we both use like us in variables in particular, they have coded every job by the occupation code and we also used the much of JSA’s analysis where they looked at the question of flexible working conditions.
The next task that we had to do was define what a Social Security job is, not immediately obvious what the cyber security sector is when you're actually looking at it. And so we collaborated with DISR and Home Affairs to build our definition of cyber security. It's a surprisingly annoying process to undertake to define what an industry is and The way we sort of narrowed it down was to look at the job titles that sort of ANZSCO had decided on and Lightcast had decide on as a cyber job and whether it contains a number of keywords. But lucky with cyber security, they love jargon. It's probably part of the problem. Like they use the word threat in a job ad. No other industry uses the word threat in a job ad slightly weird. And so we use the list of keywords essentially to identify ads, particularly useful for job ads, which is really where job titles like, I don't know, cybersecurity, the phrase penetration tester, which is a terrible piece of language or red team blue team. You might want to be like, Are we playing Halo like that [inaudible] Anyway So to get around, we use those keywords to identify what a cyber security job ad is now going through four areas where we want to measure. So we use the JSA’s analysis to see, I guess the mentions of part time and casual work, in job ads, we use Lightcast’s, proprietary algorithms to identify the number of skills listed in the job ad, essentially to understand the number of requirements used. So that's the analysis that we got free and then we analyse gendered language.
So the first way we did that is as we used an established dictionary of masculine and feminine words. So this is based on a study by Gaucher and colleagues. So what they did is they looked at masculine and feminine jobs like masculine feminine industries, looked at their job ads and using linguistics existing linguistics research, pulled out masculine words and feminine words. And what they showed in a hypothetical study was that is that women are less likely to apply for it, less likely to intend to apply for a job if they include all of these masculine words in them while they are. This is not the case for the feminine words. No, no one was worried about the feminine. But and so we used this dictionary, I guess as a baseline, which we compared to existing research.
The next step was we also used machine learning to capture, I guess, a broader list of stereotypes. The way we did that is we built on research by Burn et al who actually conducted a correspondence study which showed that using this technique you could predict discrimination in a correspondence study by identifying if there was stereotypical language used in the job. So we just picked up the technique. And so we looked we first had to train our natural language model on all English Wikipedia. So there's like million articles and you go in and get to be trained. Then we researched a list of gender stereotypes so that we can base out what we think the stereotypes are on the literature and. Then we combine those three things and we used our natural language model to measure how similar the language is in a job to stereotype, I guess like yes, a benefit of, I guess using the NLP model is it can sort of its moving away from individual words in the dictionary into phrases.
So if you're thinking about like phrase stereotypes, I guess usually exist in a phrase. So if you think about the word taking and charge. So taking charge is like this idea that men will take charge in a meeting. So that's like a masculine stereotype. But the word taking is not really and charge is not masculine at all. Like I like to charge my phone, I'm, I guess, charging into things. It doesn't really I guess it's not as like as the phrase taking charge. But what NLP allows you to do is combine all that information together to give you sort of an example of this to make it more concrete. So I stole the ad that I showed before I stole it from APS jobs. So I wanted to, I guess, add a sentence to increase the stereotype closeness, the stereotype of hackers and police. So the beautiful sentence we want a hacker skilled in the latest cybersecurity practices, ten programming languages and hacks in their free time. Just throwing in the word hack a bunch as well. That's a lot more similar to hackers in hoodie stereotype, and that raised the score by two points. So we can see it's actually quite sensitive to like a fairly stereotypical sentence. I don't think many people would really want to work for a workplace that included that sentence in their job. And there you can see that small changes can have quite a big difference. So what did we find?
First, I'll go through field requirements. So basically this is looking at the number of skills that are by occupation that they require. And with the idea once again that women are less likely to apply for a job if at lists lots of skills, as we can see in ICT, in cyber security. Are number one. We thought this would be true, but no one had actually found a good study that really showed this was the case in Australia. But yes, these two industries using love, love requiring lots and lots of skills and it puts them above well, even like lots of lots of professions, I always found like more skill sets, like engineers and scientists, which feels a little interesting for like an IT support worker that is definitely like a very high rate of requesting skills. And we can say the occupations that you expect. So like labour is what economists would say are low skilled workers or then bottom in terms of the number of actors, the average number of skills required.
Next we looked at is flexible work. This is, I think, actually our most dramatic finding. So and so this graph is kind of complicated. Break it down. So what I'm showing you at the moment is the proportion of workers who work part time hours, which is less than hours a week. We can see that essentially a lot of people who are in casual and insecure work, such as food prep assistants and hospo workers, make up the majority, have a much higher proportion of people working less than hours a week. And actually, we were actually really surprised to see this the lowest occupation working less than hours a week is actually ICT professionals with only 10% of their workforce working less than hours a week. So they're almost all working full time, which is I guess a bit surprising considering that a lot of it is white collar work where you think they'd be able to access flexible working conditions and part time. A lot of them are very big companies, they probably can afford it.
And then looking at ads is really wild. So I think focusing in on I’ll get my little pen out and focusing in on [inaudible] focusing in on ICT that tiny little bar is technically there, but only point one per cent of ICT job ads offered part time explicitly in their body text in the job ads which we were very surprised about. I think overall there is not a very good advertising of part time hours, which I was surprised about, but I was shocked to see how tiny it was. And so we don't actually have this for cyber security because at the time of the census, they didn't count cyber security as an occupation, which is kind of wild. But anyway, the ABS moves, but yeah, this tiny amount really suggests that employers are not offering explicitly part time hours. And that could be part of the reason why women are not applying for these jobs. They can't meet the characteristics of the job. They have to say if you have child caring responsibilities, you will not apply for these jobs necessarily. The next job is just this for fun. And this shows the massive increase. As David was saying in job ads offering work from home. I guess one point I want to make from this from the slide is the fact that employers can change if they want to. And so they had decided that work from home is something that they really want to compete and compete on and to make sure that they offer it. I don't have the updated numbers for this. I would love the see if it started to go down again. So we’ll have to see in the future.
Next, we’re just going to focus on masculine language, partly for brevity. We also looked feminine language, but given the masculine language is what stops women applying. This is what I'm going to present today. And so coming in, we really thought that masculine language would decrease over time. There are so many Seek articles about you can reduce gendered language, this is how you can get more women to apply focus less on masculine on like masculine language. And this is not have made any impact at all. And so the way to measure the things like websites was like gender decoder, which is based on the Gauche dictionary and we measure like this using the Gaucher dictionary and we thought that would have been some movement, partially because there has been so much media around gendered language, but there has just been no change at all, which is not good and cybersecurity is up there. We also confirmed this with the stereotype analysis. You can see even like I guess there's been a slight increase, but it kind of went a bit sort of over time seeing that there really hasn't been movement in.
So the focus on masculine stereotypes in Australia towards next we can see that cyber security is number one in caring for masculine stereotypes. They focus more on leadership ability than CEOs, which I would say is a little surprising that they want more leadership skills than managers. maybe it's not. I don't know. And they also focus a lot on problem solving and being in control, which I find a little bit confusing given they want to be in control more than security guards and people who work in prisons think cyber security is not doing well compared to the rest of the occupations in Australia and also with the validated masculine dictionary, we see that they're also number three. And I'd also like to point out that overall the ICT sector just focuses on a lot of problem solving as a key skills that they're looking for. I guess partly you could, I guess, all jobs want to solve problems, but they definitely see it as a focus area. Yeah.
Also a quick summary of this discussed. And so for ways that employers can discriminate, the employers could attract workers or attract more women to their jobs and also seen that they're currently kind of doing badly on all four areas inside the security and they would like to attract more women. They should probably change some of the language in their job ads. I just like acknowledge at the end of this talk Jobs and Skills Australia and the Department of Industry, Science and Resources and also like to say this project is a team effort. So I'd like to think and Hanne Watkins, Andrea and Scott Copely and thank you very much.
Host
Thanks Nick I can honestly say this project Sat in our team for a while now and I never get bored of hearing you present very interesting. Okay so we will now move on to our Q&A discussion with all of our presenters and we have about minutes for this section and we have a lot of questions, so I'll do my best to get through them as quickly as we can. And if you are holding on to a question in your mind, please pop it into the chat now. So we can see all of the questions and consolidate them and get them through to the panel as best we can.
But before we get started, I would like to welcome Dr. Hanne Watkins. Hanne was the project lead on the cyber job ads project that Nick just ran through, and so she'll be available to contribute to the answers for any questions directed towards this project. Okay.
Now, Liz, you've had the longest time with your mic off, so I might start with a few questions for you, if that's okay. So David and Nick can take a breather for a little while. Okay. So, Liz, knowing what you know now about the open rates for the messages in your trial, if you could go back right to the very beginning. How would you design the trial differently?
Speaker 1
Fantastic question. So thank you, Hanne. I saw your name pop up with that question. Terrific question. Short and blunt answer. I would have done a completely different trial, so I would not have done the messaging trial. I'm always within BIT I am on the voice of, Hey, let's do something other than messaging. I'm always the one kind of flying that flag. But I think this is probably a good environment for doing something that isn't a messaging trial. I probably would have worked with the platform to tangibly incentivise the workers to do something. I don't think incentivising them to slow down would actually be the right thing to do. I think that would actually probably just create a perverse incentive to game the system. I probably would have chosen a completely different target behaviour in the first place.
So essentially again, in summary, it would have been something that was not anything like what we ended up actually doing. We the caveat there and then I'll stop is that we had a lot of constraints and it's an important thing to think about when you're doing these real world trials with real companies whose priorities often lie elsewhere, that things that we want to do often morph into, things that we sort of have to do because we don't have any other choices.
Host
Right. Thank you. Now I'm someone from BETA has asked a question that I was really interested in as well. Can you tell us a little bit more about the Safari research method? And I guess in particular, is it difficult to balance the richness of the findings that you can take up from this method with the safety of the researcher involved?
Speaker 1
Yeah, another great question. So I could talk for hours about service safaris, but I won't. So service safaris are basically a classic design research methodology. So increasingly in the qualitative work we do it that we are drawing on more and more human centred design methods and this is a good example. So service safaris can be used for activities as immersive as the food delivery work all the way along to really basic things, like filling out a form or going on a website and doing a particular task the way a typical user would. So it is on the plus side, it's a great way to get an insider's view to find out what a situation is actually like when you experience it. And also early on in your research to identify what I like to think of it unknown unknowns. So things that you would never think to ask about in an interview or a survey because you simply don't know that they're a thing.
But the big caveat that I did want to draw up here is that you can't mistake your own experience in a situation for an exact replica of the actual experience that a user will have or a food delivery worker would have. So we were out there riding around doing food delivery deliveries, but we didn't experience the same level of urgency that we might if we were relying on making these deliveries to pay the bills. So we didn't get many deliveries that ultimately didn't matter. So there wasn't that same level of urgency. So our experience would be naturally different. So you do need to interpret your findings cautiously through that lens.
In terms of researcher safety, you actually need to my strong view is that you always need to consider researcher safety when you're designing any sort of experiment. So things like if you're just having people do interviews with stakeholders, thinking about their psychological safety and any sort of psychosocial impacts of interviewing stakeholders on sensitive topics or topics that might be triggering to the interviewer, it's also very important to sort of think about and also thinking about safety to the community. So you definitely need to think about that with a service safari when you have a fairly high risk activity like food delivery work. And this is actually why when we came to do the Home Care Project, we absolutely did not go any further than just looking at the WHS on boarding materials. We did not work a shift. We aren't qualified home workers. It would have posed an absolutely unacceptable risk to the recipient, to their level of care and to their privacy. It would have just been an absolute ethical minefield. So those are the things you need. Think about definitely a good thing to raise. Thanks, please.
Host
And I might just ask a quick follow on and are there any insights you can share with us about your negotiations with the partner to allow that Safari research method to take place where they open to it? Was it challenging to get them over the line?
Speaker 1
Yeah. So we it wasn't actually. So they were actually quite keen for us to experience an experience that often when you undertake this kind of work, it is as just a sort of a member of the general population. So, you know, there are some questions about whether you do need to get explicit buy in to do that or whether that actually affects the, you know, the results or the findings you might have. We actually didn't have we'd already established sort of some fairly good relationships with the key platform stakeholders in this project through interviews. So they were very open to us going in and actually doing the work ourselves.
Host
Thank you. And one last question for you, Liz, and I'll give you a break. We’re curious to know if the social media text analysis yielded, any findings that were novel or different to the interviews. Yeah, great. So the textual analysis is definitely important in helping us figure out what the priority issues were. So this is actually how we learned that verbal abuse and harassment were really top of mind and they seemed to occur quite a lot. It also gave us insights into what workers thought platforms should do differently. It's interesting because you always ask this in interviews, you say, Well, look, if whatever stakeholder you're talking about could do something differently, what would you say when you put people on the spot like that? It can be hard for them to sort of give an articulate answer. But if it's coming off of an actual experience and they're posting about it sort of in real time, you often get something a lot more detailed. So we saw a lot of posts that essentially said, I wish platforms knew X about the type of work we do or why don't platforms do Y to solve this problem. So this activity was another thing that actually helped us understand those unknown unknowns. So basically the things that we wouldn't think to ask about because we're not even aware of them in the first place. So very, very valuable from that perspective.
Host
Great. Thanks, Liz. All right, David, I might move on to you now. There's a number of questions that have come through. First up, to what extent do you think discrimination is likely to be consistent between different groups within the LGBT umbrella? So, for example, was there higher discrimination towards males and females?
Speaker 2
Yes. So we chose Australia as a sort of a queer friendly country, as a difficult environment to study. But we chose to focus on gay males because from I guess the last decade of correspondence studies, discrimination has typically been identified towards gay males, but kind of rarely towards lesbians. So in that sense we chose that particular group because that's where a lot of studies have found discrimination. In general in those correspondence studies in sort of the typical form, they found not much discrimination towards words, towards lesbians, and that's by people of either gender, towards lesbians. And in fact in some situations they've even found positive discrimination. There's one researcher, I think her name is Weichselbaumer from Austria or Germany. She did a study in Austria and a study in Germany. She actually found that in some industries there was positive discrimination towards lesbians and she identified that the reason for that was that they were less likely to give birth in the future. So it was kind of this discrimination with this idea that, well, is lower chance of having to pay out maternity benefits. So I don't really know how this would apply in our setting. It wouldn't have that same explanation because there's obviously no concerns here about its own pay on these sort of platforms. I would probably say that there would be lower discrimination, though, just because that's what's been found in past studies.
Host
Can I ask a follow up?
Speaker 2
Yes.
Host
It was great talk, David. Thank you. I was just wondering, you mentioned sort of positive discrimination. And I wondered in your study design, I thought it was really neat the way you just sort of varied whether it was a wife or a husband. But I wonder, do you think that your results could be partly driven by people preferring to do jobs for women over men? And so it's sort of more about that kind of positive discrimination towards the doing a job for the wife inside. Then like not wanting to do it for the husband of another man?
Speaker 2
So we did consider the possibility of positive discrimination when sort of designing the task, but we didn't think that that would be able to explain the proximity effects, like if you did, for instance, preferred doing jobs for women who were paying you money rather than men paying you money. It's not really clear why that would exhibit inside the house and not outside the house. Maybe you have an explanation for that. We you know, so say some explanations that or hypotheses that you could think about it to do with sort of personal safety going into a house where there are two males as opposed to not two males.
But on the other hand, these are males who don't have sexual preferences towards women workers and in fact, women in our study didn't show any discrimination at all. So it's not really clear to me how that that story would work. So I guess my hunch is that that's probably not what's going on, although I can't discount it in the data.
Host
Well, thanks. There was another related question in the chat box around the age of people who disclose their sexual preference and are they likely to be younger and therefore face more discrimination?
Speaker 2
Yeah. So that was in the in the motivation. I was talking about the stats that LGBT workers generally perform poorly compared to the rest of the population and economic indicators in most countries around the world and also in Australia. So with twice the unemployment rate, I pulled back from a study that I can't remember off the top of my head, but I remember that that study which was done in Australia, found they also broke it up by age. So if I understand correctly, when they look just at younger workers, they also found pretty much this two times difference. But there could be two things going on there.
The first thing is what I think was mentioned by Scott in the chat, which is that younger people might be more likely to identify or disclose their sexual orientation. But then the second thing is that in that older population, they might have had a bit more time, too, to find an industry that's a bit more accommodating, so a bit more time as an adult with their sexual orientation to find that their place. So you've got sort of two effects that can move in opposite directions. And the only other thing I'd say is that it's pretty consistently been found in Australia and in other places around the world that trans individuals have even worse outcomes than gay individuals of a either gender, regardless of their age. That’s well, you can try to think about designing a correspondence study where you have to signal being trans, and that's incredibly difficult to do. So that wasn't our focus. Thank you.
Host
And there's another question here around the representativeness of the study. So how representative workers in the gig economy and the participants in your study are of the general population?
Speaker 2
Yeah, I saw that study as well. I wasn't sorry - that question - I wasn't sure if that question was about how well the results apply to two discriminatory attitudes of sort of non-gig workers in the rest of the economy, or whether that was maybe going to how demographic differences amongst gig economy workers might explain some of the results versus demographic differences in the rest of the population on the first thing about generalising the results to other industries, I would kind of be hesitant to do that and just sort of say that the gig economy is important enough in and of itself at the moment with, I think we said, two thirds of Australians using it and we're finding discrimination towards users. So I would say that within the bubble of the economy, that's where our results sit.
But in terms of maybe explaining the results to do with demographic differences of the gig economy, workforce, it's actually quite difficult to find a lot of data on the demographics of typical gig economy workers. Lisa's probably got some pretty good data on this, or at least access to people have got it. But publicly it's not it's not that readily available. There was one study done, I think, by Victorian Government, Department of Premier and Cabinet in Victoria in. They found that there's a pretty even gender split, but gig economy workers were more likely to not be permanent residents or citizens more likely to be on temporary visas, which I think you pretty much expect. Within our sample. We were on a platform where in general workers there were more male workers than female workers, and it was also sort of reflected in our results. But I don't have any differences in, say, demographics across cities which could potentially be one explanation for the Melbourne results if indeed there are different demographics in gig economy workers across the cities.
Host
And that leads me to my final question, which you sort of basically addressed. Which ones do you have any thoughts against around why the Melbourne results went against those of the other capital cities?
Speaker 2
Yeah. So there are there are three explanations. First explanation is that Melbourne people are just more biased, which is unlikely to be the explanation, but it's probably the talking points. If you want to get a conversation going, I'm going to present this to the Victorian Parliament of Premier Cabinet in a few weeks and so maybe I'll just I'll let them stew on it before I give them some other hypotheses. That's unlikely to be the case. The second explanation is different demographics amongst the gig economy workforce. So there's some other variable that I currently don't have access to. I'm trying to get access to that variable, those variables to analyse it, that that might explain the differences in behaviour.
The third is kind of a very economics type argument in that it's got to do with individual decision making and uncertainty. So basically this study was run from March to July. This was after Melbourne had come out of a long lockdown and there was a small lockdown that happened during that period as well. Now why would lockdowns make people more biased? It doesn't make them more biased, according to this theory, but it makes them more risk averse. So if you're in a situation where there is high uncertainty, high risk, and you become more risk averse for some reason, you're less likely to interact in situations in which you have greater uncertainty. So if you extrapolate that and say there are types of people who you are somewhat less familiar with, you have less day to day experiences. Those are situations of higher uncertainty. You may not have any increasing bias whatsoever, but you just try to avoid uncertain situations and you still get the same results then that we find in the study. So the lockdowns could influence that without influencing the level of bias directly.
Host
Right. Great answer. Thank you. We might leave it there and head to Hanne and Nick in the room. Now start off with a nice easy one. Even I know the answer to is this study going to be published? And then I'll ask the same question of David and Liz too.
Speaker 3
So this study is going to be published, hopefully tomorrow, which is very exciting. So hopefully if everything goes well, well, it'll be up on our website tomorrow.
Host
Right. Thank You and David, I'll just give you an opportunity to comment on whether any of your research will be publicly available.
Speaker 2
Yeah, for sure. It's where we've got a working paper, but economics peer review takes a particularly annoyingly long period of time, so I'm not sure exactly when it will be published, but it would definitely will be available at some
Host
And Liz?.
Speaker 1
Yeah, all of the food delivery worker stuff is publicly available. If I can drop a link in the chat. It's all housed on the Centre for Work Health and Safety website on one page. None of the stuff from the Home Care Project is yet available. It's all been embargoed because we've got some peer reviewed manuscripts in the works, but that will become available in due course. Thanks.
Host
Thank you. And now we've got a question here, Nick and Hanne reflecting on some of the findings in the cyber job ads study relating hospitality and sales job ads that also don't appear to mention flexibility. Why do you think that may be the case?
Speaker 3
I think partly it might be driven by the fact that I see a lot of the advertising in the way that people get jobs in these industries. Is informally not necessarily responding to job ads like an online job posting. But and I imagine that the people are advertising. Are the big companies like Compass Group or things like that who are doing bulk recruitment, in which case they wouldn't Necessarily specify if it's casual or part time because a lot of those jobs would be full time in, say, hospitality Hanne any thoughts?
Speaker 4
Yeah, now that sounds reasonable. I guess the other thought I had was whether it's sort of because it's more common in those industries, you sort of feel like maybe you don't need to mention that if you're applying for a job in there or posting a job in a cafe, like of course it would just be when the cafes open or something like that. But I like your explanation better.
Host
Thank you. And I guess if you had all the policy levers available to you now, what changes would you like to make? What changes would you like employers to make in their job ads to improve the state of play?
Speaker 3
Yeah, I would love a greater increased Focus on offering flexible work and be very explicit about it and also be explicit about the fact that people in your organisation are taking flexible work. I actually as part of this, we looked at our own job ads at BETA and I notice that we don't specify that a lot of people here work part time and so I think a lot of employers could do that as a way to just broadly advertise what they're already doing. Because even though a lot of ICT workers aren’t working part time are working part time, sorry, they aren’t advertising for the people who are. Yeah, I think that's one of them. Hanne anything to add to your wish list?
Speaker 4
Start with that. No.
Host
Okay. Alright. Well, we've just about gone through all of the questions. I might just give how beautifully aligned these three studies were, I might just open up and give each of our presenters a quick opportunity to make any final comments or if you'd like to put one of the other presenters on a spot and ask your own question. I feel like this is something we don't always get to do when we're presenting our own work. Liz Can I go to you first for any final comments or any questions you'd like to throw at David, Nick or Hanne?
Speaker 1
Yeah, no questions, but just a comment. I'm really heartened by how policy focussed and practically focussed all the work that we've heard about today has been. I mean, obviously I'm biased because I work at BIT and not a university, but I think that's sort of the best part about the work that we in the behavioural science community do, is that we are obviously thinking about people in some respect because we're thinking about people's behaviour, but putting them at the heart of what we're doing and what we're aiming to achieve through the research, not just doing the research and stopping there, but thinking about how do we translate that into effective policy, effective solutions to actually create positive social impact. It's so great just to see examples of that all just peppered throughout today.
Host
Thanks, Liz. I'll go to you now. David.
Speaker 2
Yeah, I won't put my fellow speakers on the spot necessarily, but I will. I would say that with a lot of issues happening in the gig economy from experience to date, change is typically slow, slow to happen by organisations from their own initiatives. And typically the positive successes that we see come from publicity around research. So that could be academic research, that's public publicised or it can be collaborative research and reports sort that Liz is doing or it can be do it can be due to policy reports, the sort that Nick's doing.
On the latter, we haven't seen as much even in the US, we haven't seen much government led research, but I feel that’s probably the most promising way forward, actually, perhaps in collaboration with researchers. And I think that that sort of publicity, getting the big gig economy players then to sort of feel forced to get into action is probably the way forward for some of these sort of silent issues that we've discussed today.
Host
Great. Thank you. Hanne a chance to make any final comments or questions.
Speaker 4
I feel I feel very inspired to learn about these multiverse analyses and safari methods. I hadn't heard of that before. I have some more things to look into.
Host
Thank you. And Nick.
Speaker 3
Yeah. No, I don't have any comments. Just the gig Economy research is really interesting, particularly given the government's industrial relations reforms that are ongoing in moment. Yeah, but around the gig workforce.
Host
Yeah. Right. Well, thank you everyone, Liz, David, Nick and Hanne for your time today and great to see such wonderful synergy and segues take place between the work that you've presented and to see such high quality work in these spaces as others have already commented. And thank you to everyone online today. We've had over people dialling in for today's presentation, so it's wonderful to see so much interest across the BI community for this sort of work.
A quick promo the next and our final session in this the Connect series will be next Thursday, the 30th of November from 11 a.m. onwards, the focus of this session will be on being climate. So again, no shortage of ideas and research to draw from. Sorry. But you'll hear from Professor Ben Newell and Professor Michael Hiscox, who are both on BETA’s academic advisory panel, and also Saul Wodak from the Behavioural Insights Team. A colleague of Liz’s. So thank you everyone, and enjoy the rest of your afternoon.
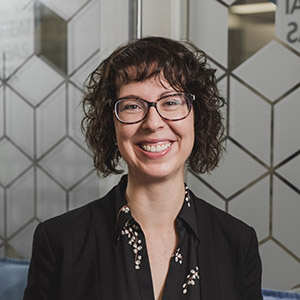
Dr Liz Convery
Dr Elizabeth (Liz) Convery is a Principal Advisor and Head of Research at the Behavioural Insights Team (BIT). In addition to leading Australia's research function, she also leads major programs of work in the areas of healthcare and work health and safety (WHS). Prior to joining BIT, Liz was a senior researcher at the National Acoustic Laboratories, where she led projects on patient-driven hearing technologies, innovative service delivery models, and a hearing aid app designed for older adults. Liz holds a PhD in Health and Rehabilitation Sciences from the University of Queensland.
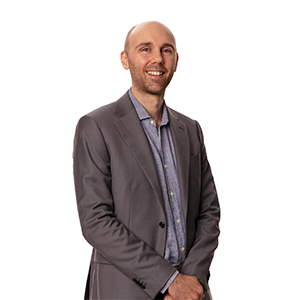
Dr David Smerdon
Dr David Smerdon is a Senior Lecturer in the University of Queensland's School of Economics. He primarily works in behavioural and development economics. His research involves theory and modelling, experiments in the lab and field, and microeconometric analysis in order to investigate topics at the intersection of these fields.
Nicholas Hilderson
Nicholas Hilderson is an advisor within BETA. Prior to BETA, Nicholas worked at the Department of Employment in behavioural economics and completed a Bachelor’s of Mathematical Science and Economics at the Australian National University (ANU). Since joining BETA, Nicholas has worked on a range of topics, from labelling for Internet of Things devices, to preventative health.