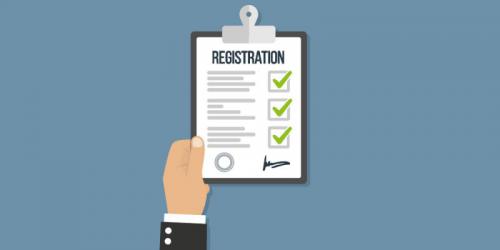
Wonk alert: This post relates to how we evaluate our behavioural–science inspired policy interventions and so is somewhat more technical than others. But there aren’t any equations!
Almost a decade ago, the replication crisis roiled psychology and then other social and biomedical sciences. The open science movement that emerged in response recommends greater transparency of hypotheses, methods of analysis, results, data, code and experimental materials (see, eg, Miguel et al 2014 and Nosek et al 2015).
Pre-registration is a key tool for greater transparency and BETA has joined this trend, aiming to pre‑register our evaluations and publish the full results whenever possible. The merits of pre‑registration have been challenged, however, in a recent paper by Aba Szollosi and others entitled Pre‑registration is redundant, at best. It thus deserves a careful and sympathetic reading from the open science community, including behavioural insights practitioners.
Szollosi and co‑authors make at least three valuable points.
First, improving psychological science (and other fields) is not just about improving methods through greater transparency—stronger theories are also crucial. Theories provide the basis for prediction and testing, and stronger theories make more predictions with clear, testable consequences. Paul Smaldino makes similar arguments in a recent article.
Second, and related, pre-registration isn’t sufficient for good science. A weak theory, transparently tested, is still a weak theory. So, in addition to rewarding transparency, it’s also worth seeking out and recognising the strength of the underlying theory, perhaps following the criteria outlined by two of the co‑authors in related papers (Szollosi and Donkin 2019a and 2019b).
Third, pre‑registration may be less necessary for testing strong theories because—by definition—a strong theory is hard to vary and thus less susceptible to HARKing (Hypothesising After the Results are Known). Ben Olken makes a similar point in the Promises and perils of pre-analysis plans, arguing that ‘[economic] theory combined with experimental design … limits the degree to which researchers can engage in data mining’ (p.76).
Ultimately, however, the co‑authors are unconvincing in their central claim that pre‑registration serves no purpose and may even be counter‑productive. The authors deny that pre‑registration addresses statistical problems associated with scientific inference, arguing that:
[statistical] problems … such as family‑wise error rates only exist when hypotheses are effectively chosen at random.
While no one chooses hypotheses at random, presumably Szollosi and co mean that a very weak theory is so flexible it allows almost any hypothesis to be chosen and hence effectively at random. By contrast, a stronger theory would ‘dictate what comparisons are necessary’ and thus act as a sufficient constraint on HARKing and inflated family‑wise error rates.
But how strong would a theory need to be to account for all the possible variations in the testing process – the choice of outcome variables and how they’re constructed, the preferred method of statistical analysis, how outliers and missing data should be handled, and so on? Even if the theory is not so strong as to dictate all such analytical choices, it could still be strong enough to generate useful inferences. And yet, without the discipline of a pre‑analysis plan, even a well-intentioned researcher might be drawn towards selectively reporting the analysis that confirms their (strong) theory, while neglecting other results.
More generally, pre‑registration is a useful bulwark—even for strong theories—against publication bias that otherwise consigns useful results (and underlying data) to the file drawer. While there may be simpler alternatives for conscientious researchers acting in good faith (eg, asking them to state whether they’ve reported all analyses), pre‑registration imposes a stricter discipline. Publication bias and selective reporting in confirmatory research are, after all, two of the main concerns that pre‑registration seeks to address (Nosek et al 2019).
Finally, while the authors are convincing in their appeal for stronger theories, how should empirical investigation proceed in the meantime? Many psychological theories are not yet formulated as mathematical models but they still impose some bounds on what constitutes confirmatory or falsifying evidence. In this context, pre‑registration seems a valuable tool to counter—at least partially—the flexibility of current theories until stronger, less flexible theories are developed.
The pre‑registration debate focuses on academic research but it also has implications for behavioural insights (BI) practitioners who seek to apply scientific findings and methods to public policy. Perhaps the case for pre-registration applies more strongly to policy impact evaluations—like ours at BETA—which test whether a government policy or program is achieving its intended objectives, rather than testing a scientific theory.
But Szollosi and his co‑authors also provide an important reminder to BI practitioners that our applications are only as good as the underlying theories we draw upon, and there is still much to be done to strengthen theories in the behavioural sciences.
I’m grateful to Aba Szollosi, Chris Donkin, Simon Gordon, Scott Copley and Tom Greenwell for comments on earlier drafts. The usual caveats apply. For more detailed discussions from both sides of the debate, see A Breakdown of “Preregistration is Redundant, at Best” by Eric-Jan Wagenmakers and Paths in strange spaces, Part 1 by Danielle Navarro.
References
Miguel, E et al (2014) ‘Promoting transparency in social science research’ Science Vol343 pp30-31 https://science.sciencemag.org/content/343/6166/30
Navarro, D (2019) ‘Paths in strange spaces, Part 1’ blog post https://djnavarro.net/post/paths-in-strange-spaces/
Nosek, B.A. et al. (2019) ‘Preregistration is hard, and worthwhile’ Trends Cogn. Sci. 23, pp815‑818 https://psyarxiv.com/wu3vs
Nosek, B et al (2015) ‘Promoting an open research culture’ Science Vol348 pp1422-1425 https://science.sciencemag.org/content/348/6242/1422.full
Olken, B (2015) ‘Promises and perils of pre-analysis plans’ Journal of Economic Perspectives 29(3) pp61-80. https://dspace.mit.edu/handle/1721.1/104069
Smaldino, P (2019) ‘Better methods can’t make up for mediocre theory’ Nature https://www.nature.com/articles/d41586-019-03350-5
Szollosi, A and Donkin, C (2019a) ‘Arrested theory development: The misguided distinction between exploratory and confirmatory research’ Preprint https://doi.org/10.31234/osf.io/suzej
Szollosi, A. and Donkin, C. (2019b) ‘Neglected Sources of Flexibility in Psychological Theories: from Replicability to Good Explanations’ Comput. Brain Behav. 2, pp190–192 https://www.researchgate.net/publication/334755580_Neglected_Sources_of_Flexibility_in_Psychological_Theories_from_Replicability_to_Good_Explanations
Szollosi, A, Kellen, D, Navarro, D, Shiffrin, R, van Rooij, I, Van Zandt, T, and Donkin, C (2019) ‘Preregistration is redundant, at best’ Preprint https://doi.org/10.31234/osf.io/x36pz
Wagenmakers, E-J (2019) ‘A Breakdown of “Preregistration is Redundant, at Best”’ blog post https://www.bayesianspectacles.org/a-breakdown-of-preregistration-is-redundant-at-best/